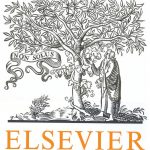
ترجمه مقاله نقش ضروری ارتباطات 6G با چشم انداز صنعت 4.0
- مبلغ: ۸۶,۰۰۰ تومان
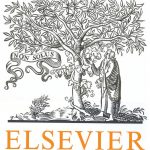
ترجمه مقاله پایداری توسعه شهری، تعدیل ساختار صنعتی و کارایی کاربری زمین
- مبلغ: ۹۱,۰۰۰ تومان
Abstract
Accurate forecasting of renewable-energy sources plays a key role in their integration into the grid. This paper proposes a novel soft computing framework using a modified clustering technique, an innovative hourly time-series classification method, a new cluster selection algorithm and a multilayer perceptron neural network (MLPNN) to increase the solar radiation forecasting accuracy. The proposed clustering method is an improved version of K-means algorithm that provides more reliable results than the K-means algorithm. The time series classification method is specifically designed for solar data to better characterize its irregularities and variations. Several different solar radiation datasets for different states of U.S. are used to evaluate the performance of the proposed forecasting model. The proposed forecasting method is also compared with the existing state-of-the-art techniques. The comparison results show the higher accuracy performance of the proposed model.
4. Conclusions
A hybrid forecasting method is developed in this paper that combines a novel clustering technique, a new hourly time-series classification method, an innovative cluster selection algorithm and MLPNNs to predict solar radiations. The silhouette algorithm is used to estimate the number of clusters. The combination of the proposed T.S.C K-means clustering and the new hourly time-series classification method provides clusters that give higher resolution in preparation for input into a MLPNN. The cluster whose data has the highest correlation with the testing data is determined by the cluster selection method to provide the training data for the solar radiation forecasting of each individual hour. The appropriate sub-train of solar radiation data as well as the temperature, wind speed, wind direction and extraterrestrial radiation data are trained by the MLPNN to provide the solar radiation forecasting result. The performance of the proposed T.S.C K-means is evaluated using several different types of datasets and compared with different variants of K-means clustering as well as K-medoids, SOM and FCM clustering algorithms. The comparison demonstrates the improved quality of the clustering for the proposed T.S.C K-means. Solar datasets with different solar characteristics and variations are used to determine the accuracy of the proposed forecasting method with T.S.C K-means. The proposed hybrid method is also compared with the existing state-of-the-art techniques for their forecast accuracies. The results show a significant accuracy improvement for the proposed forecasting model