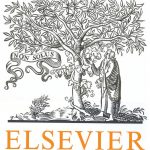
ترجمه مقاله نقش ضروری ارتباطات 6G با چشم انداز صنعت 4.0
- مبلغ: ۸۶,۰۰۰ تومان
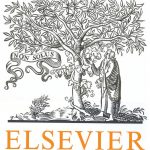
ترجمه مقاله پایداری توسعه شهری، تعدیل ساختار صنعتی و کارایی کاربری زمین
- مبلغ: ۹۱,۰۰۰ تومان
Abstract
Recently, smart grid and Energy Internet (EI) are proposed to solve energy crisis and global warming, where improved communication mechanisms are important. Softwaredefined networking (SDN) has been used in smart grid for realtime monitoring and communicating, which requires steady web-environment with no packet loss and less time delay. With the explosion of network scales, the idea of multiple controllers has been proposed, where the problem of load scheduling needs to be solved. However, some traditional load scheduling algorithms have inferior robustness under the complicated environments in smart grid, and inferior time efficiency without pre-strategy, which are hard to meet the requirement of smart grid. Therefore, we present a novel controller mind (CM) framework to implement automatic management among multiple controllers. Specially, in order to solve the problem of complexity and pre-strategy in the system, we propose a novel Quality of Service (QoS) enabled load scheduling algorithm based on reinforcement learning in this paper. Simulation results show the effectiveness of our proposed scheme in the aspects of load variation and time efficiency.
CONCLUSION AND FUTURE WORKS
In this paper, we proposed a controller mind (CM) framework to manage multiple controllers automatically and intelligently in SDEI, so as to keep the high accuracy in the real-time monitoring of smart grid. Specifically, we solved the QoS-enabled load scheduling by reinforcement learning, defined the learning agent, action space, state space, and reward function, as well leveraged the historical data to learn the load scheduling scheme offline and ahead of time, so as to realize the automatic management among multiple controllers. We simulated the performance of CM framework compared with three traditional schemes. Simulation results showed that the reinforcement learning based scheme had the best load balancing and time efficiency, which solved the problems of traditional load balancing schemes. However, the QoS-enabled load scheduling scheme learns from the historical data, so it has the lower robustness to the burst traffic. Once the burst traffic happens, state space in our scheme fails to describe all situations and also needs the longer time to learn the new allocation scheme. During this period, the load variation and time efficiency are severely affected. Future work is in progress to address these challenges.