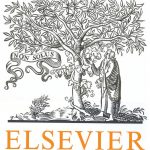
ترجمه مقاله نقش ضروری ارتباطات 6G با چشم انداز صنعت 4.0
- مبلغ: ۸۶,۰۰۰ تومان
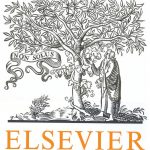
ترجمه مقاله پایداری توسعه شهری، تعدیل ساختار صنعتی و کارایی کاربری زمین
- مبلغ: ۹۱,۰۰۰ تومان
Abstract:
In this paper, to address the issue that ensembling k-nearest neighbor (kNN) classifiers with resampling approaches cannot generate component classifiers with a large diversity, we consider ensembling kNN through a multimodal perturbation-based method. Since kNN is sensitive to the input attributes, we propose a weighted heterogeneous distance Metric (WHDM). By using a WHDM and evidence theory, a progressive kNN classifier is developed. Based on a progressive kNN, the random subspace method, attribute reduction, and Bagging, a novel algorithm termed RRSB (reduced random subspace-based Bagging) is proposed for construct ensemble classifier, which can increase the diversity of component classifiers without damaging the accuracy of the component classifiers. In detail, RRSB adopts the perturbation on the learning parameter with a weighted heterogeneous distance metric, the perturbation on the input space with random subspace and attribute reduction, the perturbation on the training data with Bagging, and the perturbation on the output target of k neighbors with evidence theory. In the experimental stage, the value of k, the different perturbations on RRSB and the ensemble size are analyzed. In addition, RRSB is compared with other multimodal perturbation-based ensemble algorithms on multiple UCI data sets and a KDD data set. The results from the experiments demonstrate the effectiveness of RRSB for kNN ensembling.
6. Conclusions
In this paper, a novel multimodal perturbation-based ensemble algorithm, RRSB, is proposed, which generates accurate but diverse component classifiers to improve the performance of ensemble classification. The experimental results from multiple UCI data sets show that our proposed method can improve the classification performance in most cases. Compared with other methods, the RRSB is robust, with different k values. In addition, the testing time of RRSB is less than GA- and PSO-based methods and is comparable to FASBIR. Finally, the experimental results from the KDD Cup 99 data set show that RRSB is effective for a big and imbalanced data set. Since the output target of member classifiers only uses majority voting in this paper, in future work, we will study other combination rules on the ensemble. The study of diversity measures [41] among multimodal perturbations is clearly worthy of attention.