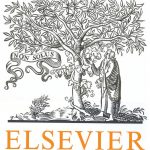
ترجمه مقاله نقش ضروری ارتباطات 6G با چشم انداز صنعت 4.0
- مبلغ: ۸۶,۰۰۰ تومان
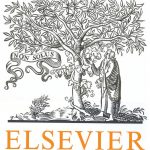
ترجمه مقاله پایداری توسعه شهری، تعدیل ساختار صنعتی و کارایی کاربری زمین
- مبلغ: ۹۱,۰۰۰ تومان
Abstract
In many real-world applications, data is collected in high dimensional spaces. However, not all dimensions are relevant for data analysis. Instead, interesting knowledge is hidden in correlated subsets of dimensions (i.e., subspaces of the original space). Detecting these correlated subspaces independently of the underlying mining task is an open research problem. It is challenging due to the exponential search space. Existing methods have tried to tackle this by utilizing Apriori search schemes. However, their worst case complexity is exponential in the number of dimensions; and even in practice they show poor scalability while missing high quality subspaces. This paper features a scalable subspace search scheme (4S), which overcomes the efficiency problem by departing from the traditional levelwise search. We propose a new generalized notion of correlated subspaces which gives way to transforming the search space to a correlation graph of dimensions. We perform a direct mining of correlated subspaces in this graph, and then, merge subspaces based on the MDL principle in order to obtain high dimensional subspaces with minimal redundancy. We theoretically show that our search scheme is more general than existing search schemes. Our empirical results reveal that 4S in practice scales near-linearly with both database size and dimensionality, and produces higher quality subspaces than state-of-the-art methods.
13. Conclusions
Mining high dimensional correlated subspaces is a very challenging but important task for knowledge discovery in multidimensional data. We have introduced 4S, a new scalable subspace search scheme that addresses the issue. 4S works in three steps: scalable computation of L2, scalable mining of Lk (k > 2), and subspace merge to reconstruct fragmented subspaces and to reduce redundancy. Our experiments show that 4S scales to data sets of more than 1.5 million records and 5000 dimensions (i.e., more than 1 trillion subspaces). Not only being more efficient than existing methods, 4S also better detects high quality correlated subspaces that are useful for outlier mining, clustering, and classification. The superior performance of 4S compared to existing methods comes from (a) our new notion of correlated subspaces that has proved to be more general than existing notions and hence, allows to discover subspaces missed by such methods, (b) our scalable subspace search scheme that can discover high dimensional correlated subspaces, and (c) our subspace merge that can recover fragmented subspaces and remove redundancy. Directions for future work include a systematic study our search scheme with different correlation measures, and the integration of the subspace merge into the correlation graph to perform an inprocess removal of redundancy