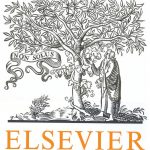
ترجمه مقاله نقش ضروری ارتباطات 6G با چشم انداز صنعت 4.0
- مبلغ: ۸۶,۰۰۰ تومان
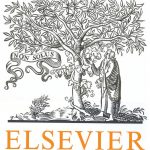
ترجمه مقاله پایداری توسعه شهری، تعدیل ساختار صنعتی و کارایی کاربری زمین
- مبلغ: ۹۱,۰۰۰ تومان
Abstract
The problem of collective classification (CC) for large-scale network data has received considerable attention in the last decade. Enabling CC usually increases accuracy when given a fully-labeled network with a large amount of labeled data. However, such labels can be difficult to obtain and learning a CC model with only a few such labels in large-scale sparsely labeled networks can lead to poor performance. In this paper, we show that leveraging the unlabeled portion of the data through semi-supervised collective classification (SSCC) is essential to achieving high performance. First, we describe a novel data-generating algorithm, called generative model with network regularization (GMNR), to exploit both labeled and unlabeled data in large-scale sparsely labeled networks. In GMNR, a network regularizer is constructed to encode the network structure information, and we apply the network regularizer to smooth the probability density functions of the generative model. Second, we extend our proposed GMNR algorithm to handle network data consisting of multi-label instances. This approach, called the multi-label regularized generative model (MRGM), includes an additional label regularizer to encode the label correlation, and we show how these smoothing regularizers can be incorporated into the objective function of the model to improve the performance of CC in multi-label setting. We then develop an optimization scheme to solve the objective function based on EM algorithm. Empirical results on several real-world network data classification tasks show that our proposed methods are better than the compared collective classification algorithms especially when labeled data is scarce.
5. Conclusions
In this paper, we first present a novel generative model with network regularization (GMNR) algorithm for semi-supervised collective classification (SSCC). For GMNR, a network regularizer encodes the network structure, and it is incorporated into the PLSA generative model to learn from network data. The resulting model provides local smoothness of the label probability distributions for classification predictions. Then, we extend the GMNR to handle the SSCC when the instances have multi-labels. The new generative model, called multi-label regularized generative model (MRGM) utilizes an additional label regularizer to explicitly encode the label correlation. The predictions of MRGM ensure consistency among interlinked instances and related labels. We evaluate the proposed GMNR and MRGM algorithms on an extensive set of real world network datasets. Empirical results show that the proposed methods perform significantly better than the other baseline collective classification methods, especially when there are only limited number of labeled data available. Future work includes the development of automated selection method for λ which controls the smoothness of our GMNR model. We will also extend the proposed methods to handle the heterogeneous network data classification problem.