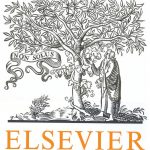
ترجمه مقاله نقش ضروری ارتباطات 6G با چشم انداز صنعت 4.0
- مبلغ: ۸۶,۰۰۰ تومان
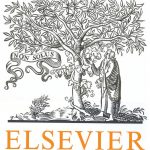
ترجمه مقاله پایداری توسعه شهری، تعدیل ساختار صنعتی و کارایی کاربری زمین
- مبلغ: ۹۱,۰۰۰ تومان
Abstract
Weak local exploitation capability of the gravitational search algorithm (GSA) and its slow convergence rate in final iterations have been demonstrated in the literature. This paper presents a modified GSA denoted here as the accelerated multi-gravitational search algorithm (AMGSA) that exhibits an improved convergence rate. In AMGSA, the simplex crossover (SPX) and the operator mutation of the breeder genetic algorithm (BGA) are incorporated with the multi-gravitational search algorithm (MGSA) to achieve an algorithm with a good exploration-exploitation balance. MGSA is adopted to prevent stagnation of the search into a local optimum (i.e. to improve the exploration capability), while the SPX and the BGA mutation operator are used to bias the search toward promising areas of the search space (i.e. to promote local exploitation). The performance of AMGSA is evaluated using several benchmark truss optimization examples. Results indicate that AMGSA not only exhibits an improved balance between the exploration and exploitation schemes but also shows competitive promise in effectively and efficiently solving large-scale optimization problems as it requires a significantly lower number of structural analyses compared to other algorithms that it is checked against.
8 Conclusions
The simplex crossover (SPX) and the operator mutation of the breeder genetic algorithm (BGA) were incorporated with the multi-gravitational search algorithm (MGSA) so as to improve its exploration and exploitation capabilities. Several benchmark truss optimization examples were used to demonstrate the efficiency of the resulting algorithm, denoted as the accelerated MGSA (AMGSA). A sensitivity analysis was carried out in order to find the best combination of the population size N, the subpopulation size Ns , and the number of the offspring created λ denoted here by the triple (N, Ns , λ) that would maximize the performance of AMGSA at a reasonable computational cost. The combination (20,10,15) was found to return an adequately good yet computationally inexpensive solution. Results of the example problems consistently showed a good exploration-exploitation balance for AMGSA and its competitive promise in effectively and efficiently solving large-scale optimization problems. This improvement was found to become more pronounced as the size of the problem increases. The BGA mutation scheme was shown to be particularly effective when the best previous positions of agents are very similar. In this case, this operator slightly changes the agents, thus improving the algorithm’s local search capability. Compared to MGSA, AMGSA offered lighter designs with considerably lower standard deviations while requiring fewer structural analyses and, therefore, less computational burden. AMGSA was further evaluated by comparing its performance with that of several other metaheuristics. Similarly, results indicated that AMGSA requires considerably fewer structural analyses to achieve the optimal solution, making the optimization process significantly faster, especially for large-scale structures.
The technique proposed in this study is not limited to the size optimization of truss structures with discrete/continuous sizing variables. Similar to the original GSA that has successfully been used in a variety of optimization problems, this technique holds promise for application in any optimization problems, including plates, shells, and frame structures subjected to static and dynamic loads. Further research, however, is required to validate its robustness and efficacy in larger scales. Additional research is also required to accurately determine the time complexity of AMGSA. We presume that, if n is the length of the string representing the population, the division of the population would require O(n) time, and the interactions among the agents would accumulate O(2n ) time, resulting in a time complexity of O(n2 n ).