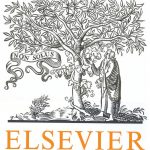
ترجمه مقاله نقش ضروری ارتباطات 6G با چشم انداز صنعت 4.0
- مبلغ: ۸۶,۰۰۰ تومان
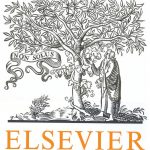
ترجمه مقاله پایداری توسعه شهری، تعدیل ساختار صنعتی و کارایی کاربری زمین
- مبلغ: ۹۱,۰۰۰ تومان
Purpose: The purpose of the research reported in this paper is to develop a quantitative model of problem solving performance of students in the computer-based mathematics learning environment. Design/methodology/approach: Regularized logistic regression was used to create a quantitative model of problem solving performance of students that predicts whether students can solve a mathematics problem correctly based on how well they solved other problems in the past. The usefulness of the model was evaluated by comparing the predicted probability of correct problem solving to the actual problem solving performance on the data set that was not used in the model building process. Findings: The regularized logistic regression model showed a better predictive power than the standard Bayesian Knowledge Tracing (BKT) model, the most frequently used quantitative model of student learning in Educational Data Mining (EDM) research. Originality/value: Providing instructional scaffolding is critical in order to facilitate student learning. However, most computer-based learning environments use heuristics or rely on the discretion of students when they determine whether instructional scaffolding needs be provided. The predictive model of problem solving performance of students can be used as a quantitative guideline that can help make a better decision on when to provide instructional supports and guidance in the computer-based learning environment, which can potentially maximize the learning outcome of students.
Conclusion
The goal of this study was to develop a quantitative model that can predict whether middle school students can solve a mathematics problem without using any hints provided in the computer-based learning environment, based on how well they solved relevant problems in the past. Although providing instructional scaffolding is critical in facilitating student learning (Koedinger and Aleven, 2007), most computer-based learning environments are using simple heuristics or relying on students when they determine whether or not instructional scaffolding needs to be provided, which is unlikely to maximize the learning outcome of students. The findings from this study may suggest that the regularized logistic regression can be used in building a quantitative model of problem solving performance of students that can help determine when to provide instructional supports and guidance to students with different abilities in the computer-based learning environment.