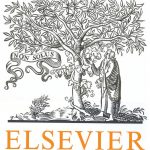
ترجمه مقاله نقش ضروری ارتباطات 6G با چشم انداز صنعت 4.0
- مبلغ: ۸۶,۰۰۰ تومان
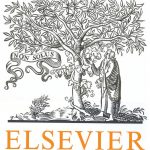
ترجمه مقاله پایداری توسعه شهری، تعدیل ساختار صنعتی و کارایی کاربری زمین
- مبلغ: ۹۱,۰۰۰ تومان
abstract
Recent developments in machine learning have expanded data-driven modelling (DDM) capabilities, allowing artificial intelligence to infer the behaviour of a system by computing and exploiting correlations between observed variables within it. Machine learning algorithms may enable the use of increasingly available ‘big data’ and assist applying ecosystem service models across scales, analysing and predicting the flows of these services to disaggregated beneficiaries. We use the Weka and ARIES software to produce two examples of DDM: firewood use in South Africa and biodiversity value in Sicily, respectively. Our South African example demonstrates that DDM (64–91% accuracy) can identify the areas where firewood use is within the top quartile with comparable accuracy as conventional modelling techniques (54–77% accuracy). The Sicilian example highlights how DDM can be made more accessible to decision makers, who show both capacity and willingness to engage with uncertainty information. Uncertainty estimates, produced as part of the DDM process, allow decision makers to determine what level of uncertainty is acceptable to them and to use their own expertise for potentially contentious decisions. We conclude that DDM has a clear role to play when modelling ecosystem services, helping produce interdisciplinary models and holistic solutions to complex socio-ecological issues.
4. Discussion
Lack of credibility, salience and legitimacy are the major reasons for the ‘implementation gap’ between ES research and its incorporation into policy- and decision-making (Clark et al., 2016; Olander et al., 2017; Wong et al., 2014). A lack of uncertainty information and the inability to run models in data-poor environments and/or under conditions where underlying processes are poorly understood may contribute to the implementation gap. However, DDM can help to address these current shortcomings in ES modelling. Here, we have demonstrated that DDM is feasible within ES science and is capable of providing estimates of uncertainty.
For our South African case study, the machine learning algorithms were able to produce a modelled output of comparable accuracy to conventional modelling methods when using the same input variables, despite our DDM using data at a much coarser (local municipality) scale (Table 3). Using the spatially attributed uncertainty (i.e., the probability of each local municipality being in Q4), decision-makers would be able to set their own level of acceptable uncertainty. In our example, since we have two categorical bins (i.e., Q1-3 and Q4), any local municipality with a modelled Q4 probability over 0.5 is assigned to the Q4 category. This assignment threshold can be varied; e.g., it is possible to state that municipalities where modelled Q4 probability is less than 0.25 or greater than 0.75 are likely to be grouped within Q1-3 and Q4 respectively, and to admit that we are less certain for the remaining municipalities. In our example, this would result in a 96% (135 out of 140) categorisation accuracy for Q1-3 and a 91% (30 out of 33) categorisation accuracy for Q4, with 53 local municipalities left uncategorised due to uncertainty.