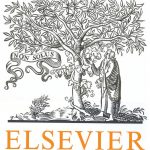
ترجمه مقاله نقش ضروری ارتباطات 6G با چشم انداز صنعت 4.0
- مبلغ: ۸۶,۰۰۰ تومان
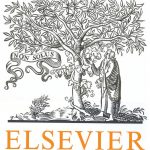
ترجمه مقاله پایداری توسعه شهری، تعدیل ساختار صنعتی و کارایی کاربری زمین
- مبلغ: ۹۱,۰۰۰ تومان
Abstract
In this work, we exploit a novel algorithm for capturing the Lie group manifold structure of the visual impression. By developing the single-layer Lie group model, we show how the representation learning algorithm can be stacked to yield a deep architecture. In addition, we design a Lie group based gradient descent algorithm to solve the learning problem of network weights. We show that our proposed technique yields representations that significantly better suited for training deep network and is also computationally efficient.
5. Conclusions
It is easier and faster for computers to recognize target objects by visual impression. This paper learns visual impression with Lie group structure during the training process of neural network by introducing the concept of Stiefel manifold. The constraint of network parameters greatly reduces the value range of parameters space, which is an outstanding advantage compared to the traditional deep learning algorithms. Experiment results further proved that Lie group impression deep learning model is a feasible method. It supplies a new approach to extract features for image recognition by deep learning methods. Based on the research results of this paper, the geometry structure of parameter space needs more in-depth research, which may help to bring a better classification performance.