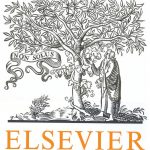
ترجمه مقاله نقش ضروری ارتباطات 6G با چشم انداز صنعت 4.0
- مبلغ: ۸۶,۰۰۰ تومان
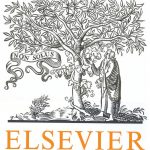
ترجمه مقاله پایداری توسعه شهری، تعدیل ساختار صنعتی و کارایی کاربری زمین
- مبلغ: ۹۱,۰۰۰ تومان
Abstract
This paper describes the integration of semantic and episodic memory (EM) models and the benefits of such integration. Semantic memory (SM) is used as a foundation of knowledge and concept learning, and is needed for the operation of any cognitive system. EM retains personal experiences stored based on their significance—it is supported by the SM, and in return, it supports SM operations. Integrated declarative memories are critical for cognitive system development, yet very little research has been done to develop their computational models. We considered structural self-organization of both semantic and episodic memories with a symbolic representation of input events. Sequences of events are stored in EM and are used to build associations in SM. We demonstrated that integration of semantic and episodic memories improves the native operation of both types of memories. Experimental results are presented to illustrate how the two memories complement each other by improving recognition, prediction, and contextbased generalization of individual memories.
VII. CONCLUSION
This paper proposed an integrated semantic and EM model. Integration improves recognition in EM by providing associated context that helps to trigger EM traces. EM influ ences activation of the SM neurons, removing ambiguities of sequential recall within a specific context. This is important in situations where the exact recall of an event is needed, rather than recall of all associated events.
An integrated declarative memory can efficiently store, consolidate, and retrieve information while considering event significance. It uses a forgetting mechanism to remove unimportant events. The model satisfies the functional requirements needed in cognitive systems and may be used to control autonomous robots using motivated reinforcement learning [18]. It is needed for symbol grounding, object recognition, concept development, cognitive understanding of perceptions, feelings, and emotions in motivated agents.
Our future work is to integrate this model with other functional blocks of the MLECOG architecture to make full use of the SM in machine learning, planning, anticipation, and thinking.