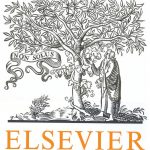
ترجمه مقاله نقش ضروری ارتباطات 6G با چشم انداز صنعت 4.0
- مبلغ: ۸۶,۰۰۰ تومان
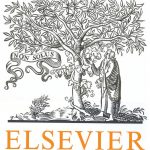
ترجمه مقاله پایداری توسعه شهری، تعدیل ساختار صنعتی و کارایی کاربری زمین
- مبلغ: ۹۱,۰۰۰ تومان
Abstract
The application of Bayesian network (BN) theory in risk assessment is an emerging trend. But in cases where data are incomplete and variables are mutually related, its application is restricted. To overcome these problems, an improved BN assessment model with parameter retrieval and decorrelation ability is proposed. First, multivariate nonlinear planning is applied to the feedback error learning of parameters. A genetic algorithm is used to learn the probability distribution of nodes that lack quantitative data. Then, based on an improved grey relational analysis that considers the correlation of variation rate, the optimal weight that characterizes the correlation is calculated and the weighted BN is improved for decorrelation. An improved risk assessment model based on the weighted BN then is built. An assessment of sea ice disaster shows that the model can be applied for risk assessment with incomplete data and variable correlation.
5 Conclusion
To deal with uncertainties including randomness and fuzziness in risk assessment, BN is applied to construct an assessment model. Risk assessment with BN is carried out according to the following process: assessment system analysis, network structure establishment, node parameter learning, and reasoning. To address the CPT calculation of qualitative nodes and correlation of variables in BN application, we did the following work: (1) The CPT calculation for qualitative nodes is transformed into a multivariate function planning problem. The error function is constructed by combining the actual assessment result. Then GA is used for CPT retrieval with error feedback searching, which achieves CPT calculation with little quantitative data; (2) The weight calculation in weighted BN is improved with GRA. We improve the GRA by introducing variation rate into the calculation of sequence difference, which enhances the correlation degree between indicators. Thus, the optimized weight from correlation is obtained and the weighted BN is improved. This research improves the application conditions of BN: big data and variable independence. In other words, the improved model can assess risk with BN in the case of incomplete data and correlated indicators. But there are also shortcomings in one respect—the optimal CPT obtained by GA must be derived from existing assessment truth, which to a certain extent limits use of the model.