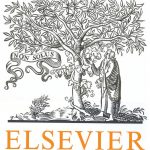
ترجمه مقاله نقش ضروری ارتباطات 6G با چشم انداز صنعت 4.0
- مبلغ: ۸۶,۰۰۰ تومان
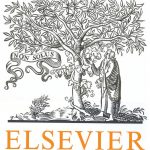
ترجمه مقاله پایداری توسعه شهری، تعدیل ساختار صنعتی و کارایی کاربری زمین
- مبلغ: ۹۱,۰۰۰ تومان
Abstract
Individuals influence each other through social interactions and marketers aim to leverage this interpersonal influence to attract new customers. It still remains a challenge to identify those customers in a social network that have the most influence on their social connections. A common approach to the influence maximization problem is to simulate influence cascades through the network based on the existence of links in the network using diffusion models. Our study contributes to the literature by evaluating these principles using real-life referral behaviour data. A new ranking metric, called Referral Rank, is introduced that builds on the game theoretic concept of the Shapley value for assigning each individual in the network a value that reflects the likelihood of referring new customers. We also explore whether these methods can be further improved by looking beyond the one-hop neighbourhood of the influencers. Experiments on a large telecommunication data set and referral data set demonstrate that using traditional simulation based methods to identify influencers in a social network can lead to suboptimal decisions as the results overestimate actual referral cascades. We also find that looking at the influence of the two-hop neighbours of the customers improves the influence spread and product adoption. Our findings suggest that companies can take two actions to improve their decision support system for identifying influential customers: (1) improve the data by incorporating data that reflects the actual referral behaviour of the customers or (2) extend the method by looking at the influence of the connections in the two-hop neighbourhood of the customers.
6. Conclusion and discussion
This study investigates an issue critical to the success of referral marketing programmes: how can a group of customers be identified who are most influential and can affect the largest number of potential customers through word-of-mouth. Previous research is generally design- and technology-oriented and use simulationbased methods to simulate influence spread over networks. Using a unique data set composed of both communication data and referral behaviour data, this study investigates whether the algorithms based on influence propagation simulations perform well in terms of identifying the most influential individuals in the network and estimating their resulting influence spread. The results show that limiting the decision support method for finding top influencers to simulations leads to overestimations of the actual influence spread and resulting product adoption. The best results are attained when referral data is used for selecting top influencers. Unfortunately, it is not that common yet for organisations to capture data about their customers’ referral behaviour. In that case, a measure of tie strength between individuals should be incorporated in the selection method as this leads to a larger influence spread. Next to that, the results also prove that it is important to not just look at the influence of the targeted customers, but also at the influence of their connections. If the connections of the most influential customers are not willing to spread word-of-mouth, there is no use in targeting these customers with a marketing campaign since the influence will not spread very far. Overall, this study shows the value of a referral behaviour detection process. A decision support system for selecting the most influential customers based on referral data allows companies to identify their most influential customers of whom the influence spread will trigger the largest cascade in product adoption. Fortunately this kind of data is becoming easier to obtain thanks to the widespread use of social media. Hence, also other organisations that possess any kind of data related to referrals or recommendations and wanting to reach a large audience can benefit from the approach suggested in this paper. In case no referral behaviour data or proxy data thereof is available, the simulation methods based on network data are already valuable and succeed in identifying influencers in a social network, although less so than those based on referral data.