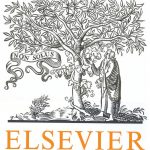
ترجمه مقاله نقش ضروری ارتباطات 6G با چشم انداز صنعت 4.0
- مبلغ: ۸۶,۰۰۰ تومان
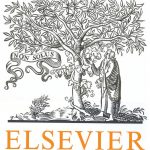
ترجمه مقاله پایداری توسعه شهری، تعدیل ساختار صنعتی و کارایی کاربری زمین
- مبلغ: ۹۱,۰۰۰ تومان
ABSTRACT
The Internet of things (IoT) has been a controversial domain of inquiry in engineering applications since the term was first introduced in 2000. The emergence of IoT also promotes the advancement of health care monitoring from face-to-face consultation to telemedicine or eHealth system. This paper presents the prototyping of an embedded health care monitoring system based on the IoT paradigm. The proposed system architecture consists of three sensors for the measurement of three basic vital signs, i.e. body temperature, pulse rate, and blood pressure. The integrated sensors are interfaced with the Intel Edison platform, and the output readings are transferred to IBM Bluemix for cloud storage and display. Taking advantage of IoT, the condition of the physical body can be monitored remotely and diagnosed with anomalies by doctors. The paper also presents the health care analytical framework related to diabetes and kidney disease. The prediction results from the analytics show the potential of the classification model to be integrated into the proposed system to identify the potential risk of certain diseases at early stages of early treatment. In the preliminary investigation, the accuracy of the developed model to differentiate between a healthy person and patients with diabetes and kidney disease is 90.54% and 87.88%, respectively. Concerning the functionality of the proposed system architecture, the sensors’ measurement accuracy is above 90% compared to conventional medical equipment.
5. CONCLUSION
In summary, an innovative framework based on IoT technology for health care monitoring applications was successfully developed. The system can perform measurements of three basic vital signs, i.e. body temperature, pulse rate, and blood pressure with high accuracy. The data is available for doctors to view and monitor in real time even if the patients perform the tests outside the hospital. Also, the study presents the machine learning framework to automatically predict the potential risks such as diabetes and kidney disease. The prediction results support further integration of the prediction model into the proposed system in next stage of works. However, the presented analytics are a preliminary investigation requiring further clinical test and real-time data to further validate the machine learning model prior to system integration. Nevertheless, due to the coupling of remote monitoring and fast analytical techniques to process the received data, it is possible to apply the described solution for health care monitoring and chronic disease control.