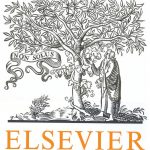
ترجمه مقاله نقش ضروری ارتباطات 6G با چشم انداز صنعت 4.0
- مبلغ: ۸۶,۰۰۰ تومان
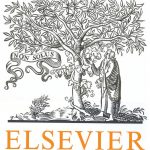
ترجمه مقاله پایداری توسعه شهری، تعدیل ساختار صنعتی و کارایی کاربری زمین
- مبلغ: ۹۱,۰۰۰ تومان
CONTENTS OF THE SPECIAL ISSUE
The first paper, entitled “Analysis of a Token Density Metric for Concern Detection in Matlab Sources using UbiSOM” is related with the MSR field. Marques et al. (2018) perform an exploratory data analysis, based on the Ubiquitous Self Organising Map (UbiSOM), to detect software concerns (i.e., cohesive set of functionalities enclosed in a module) in the Matlab language. The UbiSOM technique was illustrated using a repository with 35,000 Matlab files and the visual analytics results revealed interesting concern co-occurrence patterns. In “A Bi-objective Feature Selection Algorithm for Large Omics Datasets,” Cavique et al. (2018) propose a bi-objective version of the Logical Analysis of Inconsistent Data (LAID) algorithm. The bi-objective approach considers both the predictive accuracy and the result comprehensibility. The approach was tested using omics datasets with genome-like characteristics of patients with rare diseases, which are quite relevant for the medicine domain and that contain a high number of genetic features and very small number of samples. A huge reduction was achieved by the bi-objective approach, from one million features to just three sub-datasets with 19 features each. The third paper, “Enhancing multi-label classification for food truck recommendation,” by Rivolli et al. (2018), addresses a popular Brazilian application, food truck recommendation, which is a complex multi-label classification task. Using data from a market research and that involved hundreds of consumers, the paper explored several data-driven methods, including the proposed Ensemble of Single Label method, which reduced misclassification of under-represented labels. Vinagre et al. (2018) propose an “Online bagging for recommender systems.” The paper presents three online bagging variants for recommendation tasks in the context of data streams. Several experiments were conducted using four distinct datasets (e.g.,Movielens-1M, last.fm).