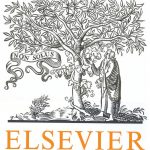
ترجمه مقاله نقش ضروری ارتباطات 6G با چشم انداز صنعت 4.0
- مبلغ: ۸۶,۰۰۰ تومان
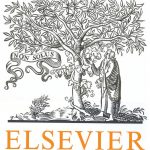
ترجمه مقاله پایداری توسعه شهری، تعدیل ساختار صنعتی و کارایی کاربری زمین
- مبلغ: ۹۱,۰۰۰ تومان
Abstract
Forecasting house price has been of great interests for macroeconomists, policy makers and investors in recent years. To improve the forecasting accuracy, this paper introduces a dynamic model averaging (DMA) method to forecast the growth rate of house prices in 30 major Chinese cities. The advantage of DMA is that this method allows both the sets of predictors (forecasting models) as well as their coefficients to change over time. Both recursive and rolling forecasting modes are applied to compare the performance of DMA with other traditional forecasting models. Furthermore, a model confidence set (MCS) test is used to statistically evaluate the forecasting efficiency of different models. The empirical results reveal that DMA generally outperforms other models, such as Bayesian model averaging (BMA), information-theoretic model averaging (ITMA) and equal-weighted averaging (EW), in both recursive and rolling forecasting modes. In addition, in recent years it is found that the Google search index, instead of fundamental macroeconomic or monetary indicators, has developed greater predictive power for house price in China.
5. Conclusions
This paper adopts the dynamic model averaging (DMA) method to predict the growth rate in house prices for 30 major cities in China. Unlike previous empirical studies, our paper uses both recursive and rolling forecasting modes in DMA. We also apply a rigorous statistical method, namely the model confidence set (MCS) test, to compare the forecasting performance of DMA with that of other models. Last, we use the Google search index as an additional predictor beyond the traditional economic variables to forecast changes in Chinese house prices. The main empirical results show that compared with the traditional time series models and other model averaging approaches, DMA is more flexible and effective, as it allows both the models and the coefficients to change over time. Under the criteria of MSFE, MAFE and many different test statistics, the MCS test indicates that DMA achieves significantly higher forecasting accuracy than other models in both the recursive and rolling forecasting modes. However, no single predictor is found to have absolute superiority over others. The best predictors for Chinese house prices vary greatly over time. Also, the Google search index for house prices has surpassed the forecasting ability of traditional macroeconomic variables in recent years.