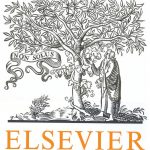
ترجمه مقاله نقش ضروری ارتباطات 6G با چشم انداز صنعت 4.0
- مبلغ: ۸۶,۰۰۰ تومان
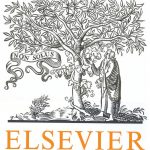
ترجمه مقاله پایداری توسعه شهری، تعدیل ساختار صنعتی و کارایی کاربری زمین
- مبلغ: ۹۱,۰۰۰ تومان
abstarct
Excess zeroes are often thought of as a cause of data over-dispersion (i.e. when the variance exceeds the mean); this claim is not entirely accurate. In actuality, excess zeroes reduce the mean of a dataset, thus inflating the dispersion index (i.e. the variance divided by the mean). While this results in an increased chance for data over-dispersion, the implication is not guaranteed. Thus, one should consider a flexible distribution that not only can account for excess zeroes, but can also address potential over- or under-dispersion. A zero-inflated Conway–Maxwell–Poisson (ZICMP) regression allows for modeling the relationship between explanatory and response variables, while capturing the effects due to excess zeroes and dispersion. This work derives the ZICMP model and illustrates its flexibility, extrapolates the corresponding likelihood ratio test for the presence of significant data dispersion, and highlights various statistical properties and model fit through several examples.
6. Discussion
This work develops a zero-inflated COM–Poisson regression to model count data containing some form of dispersion (i.e. over- or under-dispersion) and an excess number of zeroes. Such data structures appear frequently in various applications such as psychology, engineering, and business. Excess zeroes are a common cause of data over-dispersion (Hilbe, 2008). For any generated dataset, data outcomes that are zeroes add to the sample size but not to the sum total of data observations, thus diminishing the mean of the dataset. Meanwhile, these values still contribute to the variance of the dataset, thus increasing the chance that the variance is greater than the mean. However, it does not necessarily imply the overall dispersion level of a zero-inflated dataset as being overdispersed. Sellers and Shmueli (2013) provide data examples where distribution mixtures can impact the overall level of data dispersion. Because such data can be overor under-dispersed, the two-parameter COM–Poisson structure allows for more flexibility in describing the relationship between explanatory variables and the response variable—both in the count component and the zero component. In fact, we demonstrate the flexibility of the ZICMP in its ability to capture three special case zero-inflated distributions, namely the ZIP, ZIG, and logistic models. This stems from the distributional structure and statistical properties associated with the COM–Poisson distribution.