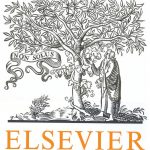
ترجمه مقاله نقش ضروری ارتباطات 6G با چشم انداز صنعت 4.0
- مبلغ: ۸۶,۰۰۰ تومان
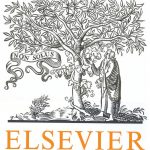
ترجمه مقاله پایداری توسعه شهری، تعدیل ساختار صنعتی و کارایی کاربری زمین
- مبلغ: ۹۱,۰۰۰ تومان
Abstract
Classification with imbalanced class distributions is a major problem in machine learning. Researchers have given considerable attention to the applications in many real-world scenarios. Although several works have utilized the area under the receiver operating characteristic (ROC) curve to select potentially optimal classifiers in imbalanced classifications, limited studies have been devoted to finding the classification threshold for testing or unknown datasets. In general, the classification threshold is simply set to 0.5, which is usually unsuitable for an imbalanced classification. In this study, we analyze the drawbacks of using ROC as the sole measure of imbalance in data classification problems. In addition, a novel framework for finding the best classification threshold is proposed. Experiments with SCOP v.1.53 data reveal that, with the default threshold set to 0.5, our proposed framework demonstrated a 20.63% improvement in terms of F-score compared with that of more commonly used methods. The findings suggest that the proposed framework is both effective and efficient. A web server and software tools are available via http://datamining.xmu.edu.cn/prht/ or http://prht.sinaapp.com/.
5. Conclusions
The disadvantage of using AUC for protein remote homology detection was explored in this study. A novel method was proposed for finding the proper prediction probability threshold of a testing set. Experimental evaluation was performed by using an established benchmark, and the results showed that the proposed method can effectively improve prediction performance over more commonly employed methods. In the future, we intend to explore the efficiency of using a function to classify a testing set, as compared with using a single threshold. We expect that a linear function will achieve better performance. Other approaches should also be employed for finding the proper prediction probability threshold, e.g., neural-like computing models [40–43], Hadoop based methods [44,45], which have widely been used in pattern recognition.