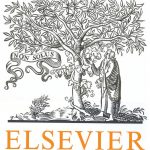
ترجمه مقاله نقش ضروری ارتباطات 6G با چشم انداز صنعت 4.0
- مبلغ: ۸۶,۰۰۰ تومان
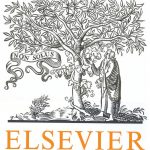
ترجمه مقاله پایداری توسعه شهری، تعدیل ساختار صنعتی و کارایی کاربری زمین
- مبلغ: ۹۱,۰۰۰ تومان
abstract
In this paper, a novel method for introducing multiplex data relationships to the SVM optimization process is presented. Different properties about the training data are encoded in graph structures, in the form of pairwise data relationships. Then, they are incorporated to the SVM optimization problem, as modified graph-regularized basekernels, each highlighting a different property about the training data. The contribution of each graph-regularized kernel to the SVM classification problem, is estimated automatically. Thereby, the solution of the proposed modified SVM optimization problem lies in a regularized space, where data similarity is expressed by a linear combination of multiple single-graph regularized kernels. The proposed method exploits and extends the findings of Multiple Kernel Learning and graph-based SVM method families. It is shown that the available kernel options for the former can be broadened, and the exhaustive parameter tuning for the latter can be eliminated. Moreover, both method families can be considered as special cases of the proposed formulation, hereafter. Our experimental evaluation in visual data classification problems denote the superiority of the proposed method. The obtained classification performance gains can be explained by the exploitation of multiplex data relationships, during the classifier optimization process.
5. Conclusion
We have presented a novel method for introducing multiplex data relationships to the SVM optimization process, by exploiting pairwise data information expressed in multiple graph structures. Our experiments denoted that the proposed method provided consistently increased classification performance against the competition, in different visual data classification problems. The improved classification accuracy was mainly achieved, due to the exploitation of advanced graph-based regularization settings in an optimal fashion, effectively representing the multimodal/multiplex image and video data characteristics. Since the proposed method provided enhanced classification performance using various descriptor settings, including simple pixel luminosities, advanced handcrafted feature types and deep representations, it should be expected that it will perform well in other standard classification problems, as well. Moreover, since the proposed method is a generic formulation for Graph-based SVM methods and Multiple Kernel methods, evolution in both fields shall favor the proposed method as well. That is, novel advanced regularization settings using graph types unknown at the present, perhaps exploiting deep learning architectures, could be integrated with the proposed formulations. In addition, advanced Multiple Kernel Learning solvers that will be introduced in the future can be employed for solving the proposed optimization problem. Evolution in both domains can serve as a feature research direction.