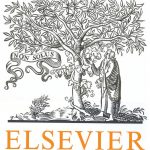
ترجمه مقاله نقش ضروری ارتباطات 6G با چشم انداز صنعت 4.0
- مبلغ: ۸۶,۰۰۰ تومان
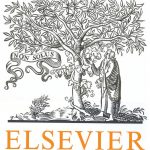
ترجمه مقاله پایداری توسعه شهری، تعدیل ساختار صنعتی و کارایی کاربری زمین
- مبلغ: ۹۱,۰۰۰ تومان
Abstract
Clustering is performed to get insights into the data whose volume makes it problematic for analysis by humans. Due to this, clustering algorithms have emerged as meta learning tools for performing exploratory data analysis. A Cluster is defined as a set of objects which have a higher degree of similarity to each other compared to objects not in the same set. However there is ambiguity regarding a suitable similarity metric for clustering. Multiple measures have been proposed related to quantifying similarity such as euclidean distance, density in data space etc. making clustering a multi-objective optimization problem. In this paper, different clustering approaches are studied from the theoretical perspective to understand their relevance in context of massive data-sets and empirically these have been tested on artificial benchmarks to highlight their strengths and weaknesses.
4. Conclusion
Cluster detection poses a challenge to algorithms especially when underlying model for formation of community structure is not available. This is the case in most real world situations and hence there is ambiguity regarding defining the term ”cluster”. Ideally the approach to clustering should not require user interference, however all the current clustering algorithms require parameter tuning and this could result in models that over-fit the data and don’t generalize well. The algorithms could not identify clusters in the benchmark data-sets and had drawbacks like sensitivity to noise and outliers, high time and computational complexity and failure to detect clusters which were not well separated or of arbitrary shapes and densities.