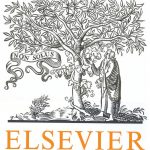
ترجمه مقاله نقش ضروری ارتباطات 6G با چشم انداز صنعت 4.0
- مبلغ: ۸۶,۰۰۰ تومان
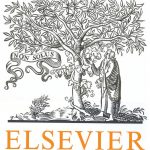
ترجمه مقاله پایداری توسعه شهری، تعدیل ساختار صنعتی و کارایی کاربری زمین
- مبلغ: ۹۱,۰۰۰ تومان
Abstract
In e-learning, most content-based (CB) recommender systems provide recommendations depending on matching rules between learners and learning objects (LOs). Such learner-oriented approaches are limited when it comes to detecting learners’ changes, furthermore, the recommendations show low adaptability and diversity. In this study, in order to improve the adaptability and diversity of recommendations, we incorporate an LO-oriented recommendation mechanism to learner-oriented recommender systems, and propose an LO self-organization based recommendation approach (Self). LO self-organization means LO interacts with each other in a spontaneous and autonomous way. Such self-organization behavior is conducive to generating a stable LO structure through information propagation. The proposed approach works as follows: firstly, LOs are simulated as intelligent entities using the self-organization theory. LOs can receive information, transmit information, as well as move. Secondly, an environment perception module is designed. This module can capture and perceive learner’s preference drifts by analyzing LOs’ self-organization behaviors. Finally, according to learners’ explicit requirements and implicit preference drifts, recommendations are generated through LOs’ self-organization behaviors. Based on application to real-life learning processes, the ample experimental results demonstrate the high adaptability, diversity, and personalization of the recommendations.
Conclusions and Future Work
The importance of improving the adaptability and diversity of recommender systems has strongly 705 emerged. The fast-changing characteristics of the e-learning environment show a higher demand for adaptability and diversity than in other fields. In this paper, we propose an LO’s self-organization based recommendation approach in e-learning. The LO-oriented recommender mechanism is combined with the learner-oriented CB recommender system. LOs are modeled as intelligent entities, and related metadata are extended to describe the LO’s state. To ensure the intelligent recommendations, we propose 710 a bottom-up and distributed self-organization recommendation strategy. With the stimuli of the learner’s behaviors, LOs interacts with other LOs in an autonomous way. The positive information carried by LOLO relationships is a critical criterion for LOs’ behaviors. Moreover, the environment perception module is designed to make adaptive and predictive recommendations by analyzing both learners’ learning activities and LOs’ self-organization behavior. All of the experimental results indicate that the proposed approach 715 can increase the possibility of diversity and adaptability in terms of little cost of precision. The definitive characteristics of the self-organization recommendation approach can be summarized as follows: excellent personalized performance, degradation of excessive recommendations, improvement of diversity, and good adaptability performance. In future work, more case studies will be used to verify the effectiveness of the proposed approach, 720 especially in massive open online courses (MOOCs). Furthermore, we will study the recommender systems based on learners’ self-organization behaviors, Finally, we will devote to finding a way to combine LO self-organization and learner self-organization to provide personalized recommendations is also a key issue.