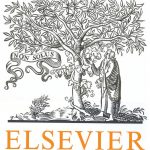
ترجمه مقاله نقش ضروری ارتباطات 6G با چشم انداز صنعت 4.0
- مبلغ: ۸۶,۰۰۰ تومان
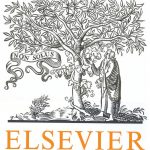
ترجمه مقاله پایداری توسعه شهری، تعدیل ساختار صنعتی و کارایی کاربری زمین
- مبلغ: ۹۱,۰۰۰ تومان
Abstract
Along with the rapid growth of computer networks comes the need for automating management functions to prevent errors in decision-making and reduce the cost of ordinary operations. Software-defined networking (SDN) is an emergent paradigm that aims to support next-generation networks through its flexible and powerful management mechanisms. Although SDN provides greater control over traffic flow, its security and availability remain a challenge. The major contribution of this paper is to present an SDN-based ecosystem that monitors network traffic and proactively detects anomalies which may impair proper network functioning. When an anomalous event is recognized, the proposal conducts a more active analysis to inspect irregularities at the network traffic flow level. Detecting such problems quickly is essential to take appropriate countermeasures. In this manner, the potential for centralized network monitoring based on SDN with OpenFlow is addressed in order to evaluate mitigation policies against threats. Experimental results demonstrate the proposed ecosystem succeeds in achieving higher detection rates compared to other approaches. In addition, the performance analysis shows that our approach can efficiently contribute to the network’s resilience.
Conclusion
This work presented an ecosystem designed to detect and mitigate network threats in an SDN environment. The system autonomously monitors the traffic and implements countermeasures on affected devices to maintain the availability of the network’s services. To this end, the system employs a multi-feature traffic analysis to profile normal network usage. Thereafter, the resulting normal profile is used to identify unusual traffic patterns, and a mitigation policy is chosen according to the recognized anomalies. The ecosystem was evaluated using a testbed simulating DDoS and port scan attacks. Compared to other anomaly detection approaches, our detection mechanism proved outstanding in terms of accuracy and low false-positive rate. The system locates interfaces that attackers have compromised. The mitigation module uses this information to begin mitigation routines at the affected switches and, therefore, restores proper network functionality. Regarding performance, we evaluated our proposed system’s performance concerning execution time and system resources usage (controller’s CPU and switches flow table size). This analysis demonstrated that the detection strategy did not vary significantly concerning the type of recognized anomaly as there is no need to retrain if we apply the MLR approach. In contrast, the more intense the attack, the more time was spent to execute the mitigation and preprocessing routines. However, even under high attack intensity, the modules remain operative, and they perform in milliseconds. By evaluating the controller’s CPU usage and the number of active flows in the switches, it was possible to observe the effectiveness of the policies for preventing the attacks from spreading. The results indicated that the proposed mechanism could quickly start the attack detection and prescribe countermeasures to the switches, although it requires some time to recognize a DDoS attack. The ecosystem presents a modular design, as statistic collection, anomaly detection, mitigation, and report tasks are decoupled and can conveniently be adapted to address new security issues. In this manner, in future work, we intend to study and incorporate into our ecosystem for SDN networks new anomaly detection techniques as well as corresponding mitigation policies. We will also extend this work to consider zero-day attacks that may compromise proper SDN operations.