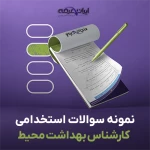
سوالات استخدامی کارشناس بهداشت محیط با جواب
- مبلغ: ۸۴,۰۰۰ تومان
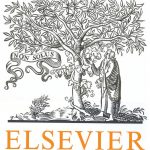
ترجمه مقاله نقش ضروری ارتباطات 6G با چشم انداز صنعت 4.0
- مبلغ: ۸۶,۰۰۰ تومان
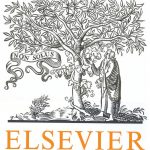
ترجمه مقاله پایداری توسعه شهری، تعدیل ساختار صنعتی و کارایی کاربری زمین
- مبلغ: ۹۱,۰۰۰ تومان
Advances in biological and medical technologies have been providing us explosive volumes of biological and physiological data, such as medical images, electroencephalography, genomic and protein sequences. Learning from these data facilitates the understanding of human health and disease. Developed from artificial neural networks, deep learning-based algorithms show great promise in extracting features and learning patterns from complex data. The aim of this paper is to provide an overview of deep learning techniques and some of the state-of-the-art applications in the biomedical field. We first introduce the development of artificial neural network and deep learning. We then describe two main components of deep learning, i.e., deep learning architectures and model optimization. Subsequently, some examples are demonstrated for deep learning applications, including medical image classification, genomic sequence analysis, as well as protein structure classification and prediction. Finally, we offer our perspectives for the future directions in the field of deep learning.
Introduction
Deep learning is a recent and fast-growing field of machine learning. It attempts to model abstraction from large-scale data by employing multi-layered deep neural networks (DNNs), thus making sense of data such as images, sounds, and texts [1]. Deep learning in general has two properties: (1) multiple layers of nonlinear processing units, and (2) supervised or unsupervised learning of feature presentations on each layer [1]. The early framework for deep learning was built on artificial neural networks (ANNs) in the 1980s [2], while the real impact of deep learning became apparent in 2006 [3,4]. Since then, deep learning has been applied to a wide range of fields, including automatic speech recognition, image recognition, natural language processing, drug discovery, and bioinformatics [5–7].
The past decades have witnessed a massive growth in biomedical data, such as genomic sequences, protein structures, and medical images, due to the advances of highthroughput technologies. This deluge of biomedical big data necessitates effective and efficient computational tools to store, analyze, and interpret such data [5,8]. Deep learning-based algorithmic frameworks shed light on these challenging problems. The aim of this paper is to provide the bioinformatics and biomedical informatics community an overview of deep learning techniques and some of the state-of-the-art applications of deep learning in the biomedical field. We hope this paper will provide readers an overview of deep learning, and how it can be used for analyzing biomedical data.
Medical image classification and segmentation
Machine learning for medical images has long been a powerful tool in the diagnosis or assessment of diseases. Traditionally, discriminative features referring to medical image interpretation are manually designed for classification (detection of lesions or abnormalities) and segmentation of regions of interest (tissues and organs) in different medical applications. This requires the participation of physicians with expertise. Nonetheless, the complexity and ambiguity of medical images, limited knowledge for medical image interpretation, and the requirement of large amounts of annotated data have hindered the wide use of machine learning in the medical image domain. Notably, deep learning methods have attained success in a variety of computer vision tasks such as object recognition, localization, and segmentation in natural images. These have soon brought about an active field of machine learning in medical image analysis.
پیشرفت های انتزاعی در زیست و تکنولوژی های زیستی، حجم بسیار زیادی از داده های زیستی و فیزیولوژیک را ایجاد کرده است، از جمله تصویر های پزشکی، الکتروانسفالوگرافی، نقشه های ژنوم و توالی های پروتئینی. یادگیری با استفاده از این داده ها منجر به تسهیل درک ما نسبت به سلامت و بیماری های انسان می شود. الگوریتم های مبتنی بر یادگیری عمیق که از شبکه های عصبی مصنوعی توسعه پیدا کرده اند، توانایی بالایی برای استخراج کردن ویژگی و الگوهای یادگیری از داده های پیچیده را از خودشان نشان داده اند. هدف این مقاله فراهم کردن مروری بر روی تکنیک های یادگیری عمیق و بعضی از جدید ترین کاربرد های آن ها در زمینه ی پزشکی می باشد. ما نخست توسعه ی شبکه های عصبی و یادگیری عمیق را ارائه می کنیم. سپس دو بخش اصلی از یادگیری عمیق، یعنی معماری یادگیری عمیق و بهینه سازی مدل را ارائه می کنیم. در نهایت بعضی از نمونه های کاربردی روش یادگیری عمیق ارائه می گردد ، از جمله طبقه بندی تصاویر پزشکی، تحلیل توالی ها، و همچنین طبقه بندی و پیش بینی کردن ساختار پروتئین ها. در نهایت، ما دیدگاه خودمان را در رابطه با جهت آتی در زمینه ی یادگیری عمیق را ارائه می کنیم.
مقدمه
یادگیری عمیق یکی از روش های جدید با رشد سریع در زمینه ی یادگیری ماشین می باشد. در این روش تلاش می شود تا استخراج ویژگی ها و اطلاعات مفید از داده هایی با مقیاس بزرگ، با استفاده از شبکه های عصبی عمیق چند لایه ای (DNN ها) ، استخراج شود تا بتوان از داده هایی مانند تصاویر، صداها و متن ها، اطلاعات مفید به دست آورد [1]. یادگیری عمیق به صورت عمومی دارای دو ویژگی می باشد : 1)لایه های چندگانه از واحد های پردازش غیر خطی و 2) یادگیری با سرپرست یا بدون سرپرست از نمایه ی ویژگی ها در هر لایه [1].قالب کاری اولیه برای یادگیری عمیق بر اساس شبکه های عصبی مصنوعی (ANN ها) در دهه ی 1980 ایجاد شد [2] ، در حالی که تاثیر واقعی این روش های یادگیری عمیق در سال 2006 نمایان شد [3و4]. از آن زمان تا کنون، یادگیری عمیق در گستره ی زمینه های مختلف، شامل شناسایی خودکار گفتار، شناسایی تصویر، پردازش طبیعی زبان، شناسایی دارو و انفورماتیک زیستی مورد استفاده قرار گرفته است [5-7].
در دهه های اخیر، رشد گسترده ای در زمینه ی داده های پزشکی مانند توالی های ژنی، ساختار پروتئین ها، و تصویر های پزشکی ایجاد شده است که به دلیل پیشرفت در تکنولوژی هایی با کارایی بالا بوده است. این طوفان گسترده از داده های بزرگ زیستی، منجر به الزام استفاده از ابزار محاسباتی موثر و کارآمد شده است تا بتوانیم این چنین داده هایی را ذخیره سازی، تحلیل و تفسیر کنیم. قالب های کاری یادگیری عمیق ، مسائل جدیدی را نمایان کرده اند. هدف این مقاله فراهم کردن یک مرور در رابطه با یادگیری عمیق و بعضی از جدید ترین کاربرد های یادگیری عمیق در زمینه ی پزشکی و انفورماتیک زیستی می باشد.ما امید داریم که این مقاله بتواند برای خوانندگان ، مروری مناسب در رابطه با یادگیری عمیق ایجاد کرده و کاربرد های آن برای تحلیل داده های زیستی را نمایان کند.
طبقه بندی تصویر های پزشکی و بخش بندی آن ها
یادگیری ماشینی برای تصویر های پزشکی ، یک ابزار قوی در تشخیص و یا ارزیابی بیماری ها بوده است. معمولا، ویزگی های متمایز اشاره به تفسیر تصویر های پزشکی دارد که معمولا پیش از این، به صورت دستی توسط انسان ها برای طبقه بندی ( شناسایی کردن آسیب ها یا حالت های غیر عادی) و یا بخش بندی منطقه های مد نظر ( بافت ها و ارگان ها) در زمینه های پزشکی مختلف، مورد استفاده قرار می گرفته است. این روند نیازمند مشارکت متخصص ها و پزشکان می باشد. در هر صورت، پیچدگی و ابهام تصویر های پزشکی، دانش محدود برای تفسیر تصاویر پزشکی و الزام مقادیر داده های تفسیری مانع استفاده ی گسترده از یادگیری ماشینی در دامنه ی تصویر های پزشکی شده است. اما باید به این نکته اشاره کرد که روش های یادگیری عمیق ، موفقیت زیادی در انواع وظایف بصری کامپیوتری مانند شناسایی اشیا، مشخص کردن مکان آن ها و یا بخش بندی تصویر های طبیعی به دست آورده است. این موارد ، باعث شده که این روش ها از نظر تفسیر تصویر های پزشکی نیز امید بخش باشند.