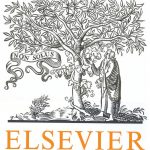
ترجمه مقاله نقش ضروری ارتباطات 6G با چشم انداز صنعت 4.0
- مبلغ: ۸۶,۰۰۰ تومان
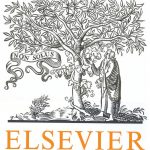
ترجمه مقاله پایداری توسعه شهری، تعدیل ساختار صنعتی و کارایی کاربری زمین
- مبلغ: ۹۱,۰۰۰ تومان
ABSTRACT
The systematic study of nuclei patterns in pathology images is very important for fully charactering the grade of cancerous tissues. Nowadays, with the great advance of deep neural networks (i.e., DNN), intense interest in adopting DNN to distinguish different types of pathology nuclei is widely spread. However, most of the existing methods need to annotate lots of nuclei images in the training stage, and this is not always an option for the labelling cost are high. To address this problem, we propose a novel approach called DAPC (i.e., deep active learning with pairwise constraints) to actively select the most valuable nuclei for annotation. Specifically, we firstly design a novel pairwise-constraint regularized deep convolutional neural network (i.e., CNN) that can simultaneously preserve the distribution of different subjects and optimize the objective criterion of conventional CNN. Then, through the properly designed CNN, we query the most informative nuclei in the unlabelled dataset for human annotation, and the parameters of the designed CNN is subsequently updated by incorporating the newly annotated samples to enhance the CNNs performance incrementally. We evaluate our method on a public available pathology colon dataset, the experimental results show that the proposed method could achieves to a weighted F1-score of 79.2% by only annotating 60% nuclei in the training set, which is better than the comparing methods.
4. CONCLUSION
In this paper, we propose an interactive cell nuclei classification method (i.e., DAPC). The best merit of our approach is its capability of reducing the workload of expert for annotation. The experimental results on a public available colon dataset demonstrate that our method can save experts 40% time for annotation while still can achieve better classification performance when comparing with several existing methods.