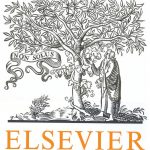
ترجمه مقاله نقش ضروری ارتباطات 6G با چشم انداز صنعت 4.0
- مبلغ: ۸۶,۰۰۰ تومان
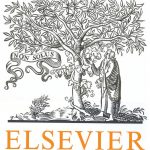
ترجمه مقاله پایداری توسعه شهری، تعدیل ساختار صنعتی و کارایی کاربری زمین
- مبلغ: ۹۱,۰۰۰ تومان
ABSTRACT
Renal cell cancer is the most common type of kidney cancer and usually occurs at an advanced ages. The rapid spread of renal cell cancer and the inability to detect the disease early often results in a fatality. Therefore, it is important to identify the renal abnormalities before the disease reaches the advanced phase. This paper proposes a decision support system that detects renal cell cancer using abdominal images of healthy and renal cell cancer tissues. Renal cell cancer detection involves two main stages as segmentation and cancer detection. In the first step, the kidney areas have been obtained by segmentation based on clustering analysis. In the second step, classification has been made by computer-assisted detection system to identify renal cell cancer. Feature vectors that support the originality of the study at this stage have been created. Subsequently, classification has been made using these feature vectors with the Support Vector Machines (SVMs). For detecting the renal abnormality, 130 different images obtained from the image archiving system of the Radiodiagnostic Department of Fırat University Medical Faculty were used. Thirty of these images have been used to train the K-means classifier. Performance evaluations have been made for both segmentation and classification. In order to measure segmentation success, the Dice coefficient was obtained as 89.3%. Sensitivity, Specificity, Accuracy, Positive Predictive Value (PPV) and Negative Predictive Value (NPV) coefficients, which have been used to determine the classification performance, were obtained as 84%, 92%, 88%, 91.3% and 85.19% respectively.
5. CONCLUSION
The first goal of the paper is to automatically perform kidney segmentation on abdominal CT images. The second goal is to detect renal cell cancer tissue from those images. A decision support system has been proposed in this article to achieve the stated objectives. One of the most important features of the decision support system is the specified attribute vectors. To construct the feature vectors, we obtained the attributes after the segmentation of kidneys as the total pixel values of the right and left kidneys, mean density values of the right and left kidneys, and the area and density ratios of both kidneys. Firstly, right and left kidneys were segmented from the images. In the segmentation process, the spinal cord was taken as a reference, unlike in previous studies. For the performance assesment of the proposed segmentation methodology, the Dice criterion was used and average 89.3% segmentation success was achieved. At the end of this process, a second data set (feature vectors) was created from the segmented kidneys for the diagnostic support system. A Support Vector Machine was used for classification of renal cell cancer in the diagnostic support system. The SVM was tested on 100 CT images. Sensitivity, Specificity, Accuracy, PPV and NPV criteria were used for the performance of the decision support system. These values were obtained as 84%, 88%, 92%, 91.3% and 85.19% respectively. The results were checked by the doctor and determined to be reasonable. There are many criteria that influence the performance of the proposed decision support system. The most important and decisive factor of these criteria is to make the correct segmentation. This results are obtained from the presence of many organs on the abdominal images, which makes it difficult to isolate the kidneys. We anticipate that this work will shed light on the future work so that the performance values are higher.