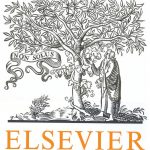
ترجمه مقاله نقش ضروری ارتباطات 6G با چشم انداز صنعت 4.0
- مبلغ: ۸۶,۰۰۰ تومان
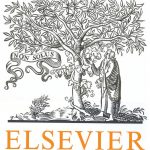
ترجمه مقاله پایداری توسعه شهری، تعدیل ساختار صنعتی و کارایی کاربری زمین
- مبلغ: ۹۱,۰۰۰ تومان
ABSTRACT
We propose and test three different probabilistic classification techniques using data envelopment analysis (DEA). The first two techniques assume parametric exponential and half-normal inefficiency probability distributions. The third technique uses a hybrid DEA and probabilistic neural network approach. We test the proposed methods using simulated and real-world datasets. We compare them with cost-sensitive support vector machines and traditional probabilistic classifiers that minimize Bayesian misclassification cost risk. The results of our experiments indicate that the hybrid approach performs as well as or better than other techniques when misclassification costs are asymmetric. The performance of exponential inefficiency distribution DEA classifiers is similar or better than that of traditional probabilistic neural networks. We illustrate that there are certain classification problems where probabilistic DEA based classifiers may provide superior performance compared to competing classification techniques.
5. Summary, discussion, and directions for future work
Our study indicates that probabilistic DEA techniques may hold the promise of improved classification results in certain focused classification problem domains. This DEA classification niche requires a linearly inseparable classification problem with continuous decisionmaking attributes. Additionally, monotonicity, where higher values of decision-making attributes lead to classification into the class label of 1, and exponential class distributions may be desired. Generally, the DEAPNN technique performs as well as or better than competing misclassification cost-sensitive SVM. However, the DEA-PNN can select too few examples to learn its class distribution PDFs when training data sample sizes are small (fewer than 100 examples). Using traditional PNN as a benchmark allows a decision-maker to detect if training sample sizes are too small, and may, therefore, impact the performance of DEA-PNN. Thus, a decision-maker may use DEA-PNN and PNN results together and improve classification accuracy by making adjustments to the DEA-PNN threshold so that the results it produces are always better than or equal to those of the PNN.
In a classification problem where all decision-making variables are categorical, the DEA technique should not be used. However, for mixed decision-making attributes, where some variables are continuous and others are categorical, a few modifications can be made to incorporate categorical variables. First, if these categorical variables are ordinal, then Banker and Morey’s (1986) non-controllable models can be used to incorporate them along with other continuous variables. If all categorical variables are non-ordinal and binary, then these binary variables can be relabeled using a methodology that is similar to the class relabeling described in Section 3. For categorical variables that are neither binary nor ordinal, training data needs to be split for each category of the variable, and separate DEA models may be built for each category. Such an analysis is combinatorial in nature and requires large size datasets so that training datasets for each category have linear inseparable classification problems. In practice, non-ordinal categorical variables, along with continuous variables, may impose a limit on the use of DEA models for classification problems. For such problems, a better approach may be to use hybrid techniques that process categorical variables and continuous variables separately. Perhaps use of decision trees for categorical variables, and DEA for continuous variables may be an option for such a hybrid technique. Future research is needed in this area.