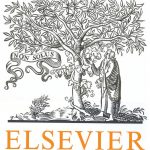
ترجمه مقاله نقش ضروری ارتباطات 6G با چشم انداز صنعت 4.0
- مبلغ: ۸۶,۰۰۰ تومان
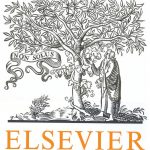
ترجمه مقاله پایداری توسعه شهری، تعدیل ساختار صنعتی و کارایی کاربری زمین
- مبلغ: ۹۱,۰۰۰ تومان
Abstract
Seizure prediction has attracted growing attention as one of the most challenging predictive data analysis efforts to improve the life of patients with drug-resistant epilepsy and tonic seizures. Many outstanding studies have reported great results in providing sensible indirect (warning systems) or direct (interactive neural stimulation) control over refractory seizures, some of which achieved high performance. However, to achieve high sensitivity and a low false prediction rate, many of these studies relied on handcraft feature extraction and/or tailored feature extraction, which is performed for each patient independently. This approach, however, is not generalizable, and requires significant modifications for each new patient within a new dataset. In this article, we apply convolutional neural networks to different intracranial and scalp electroencephalogram (EEG) datasets and propose a generalized retrospective and patient-specific seizure prediction method. We use the short-time Fourier transform on 30-s EEG windows to extract information in both the frequency domain and the time domain. The algorithm automatically generates optimized features for each patient to best classify preictal and interictal segments. The method can be applied to any other patient from any dataset without the need for manual feature extraction. The proposed approach achieves sensitivity of 81.4%, 81.2%, and 75% and a false prediction rate of 0.06/h, 0.16/h, and 0.21/h on the Freiburg Hospital intracranial EEG dataset, the Boston Children’s Hospital–MIT scalp EEG dataset, and the American Epilepsy Society Seizure Prediction Challenge dataset, respectively. Our prediction method is also statistically better than an unspecific random predictor for most of the patients in all three datasets.
Conclusion
Seizure prediction capability has been studied and improved over the last four decades. A perfect prediction is not yet available, but with current prediction 345 performance it appears possible to provide patients with a warning so they can take some precautions for their safety. We proposed a novel approach of using CNNs with minimum feature engineering. The proposed approach showed its good generalization in working well with both iEEG and sEEG data. This gives more patients the opportunity to possess a seizure prediction device that can 350 help them have a more manageable life.