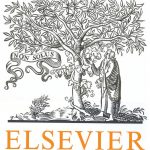
ترجمه مقاله نقش ضروری ارتباطات 6G با چشم انداز صنعت 4.0
- مبلغ: ۸۶,۰۰۰ تومان
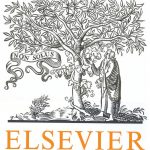
ترجمه مقاله پایداری توسعه شهری، تعدیل ساختار صنعتی و کارایی کاربری زمین
- مبلغ: ۹۱,۰۰۰ تومان
Abstract
Due to the growing needs of motion capture (mocap) in movie, video games, sports, etc., it is highly desired to compress mocap data for efficient storage and transmission. Unfortunately, the existing compression methods have either high latency or poor compression performance, making them less appealing for time-critical applications and/or network with limited bandwidth. This paper presents two efficient methods to compress mocap data with low latency. The first method processes the data in a frame-by-frame manner so that it is ideal for mocap data streaming. The second one is clip-oriented and provides a flexible trade-off between latency and compression performance. It can achieve higher compression performance while keeping the latency fairly low and controllable. Observing that mocap data exhibits some unique spatial characteristics, we learn an orthogonal transform to reduce the spatial redundancy. We formulate the learning problem as the least square of reconstruction error regularized by orthogonality and sparsity, and solve it via alternating iteration. We also adopt a predictive coding and temporal DCT for temporal decorrelation in the frame- and clip-oriented methods, respectively. Experimental results show that the proposed methods can produce higher compression performance at lower computational cost and latency than the state-of-the-art methods. Moreover, our methods are general and applicable to various types of mocap data.
6. Conclusion
We presented frame- and clip-based methods for compressing mocap data with low latency. Taking advantage of the unique spatial characteristics, we proposed learned spatial decorrelation transform to effectively reduce the spatial redundancy in mocap data. Due to its data adaptive nature, LSDT outperforms the commonly used data-independent transforms, such as discrete cosine transform and discrete wavelet transform, in terms of the decorrelation performance. Experimental results show that the proposed methods can produce higher compression ratios at a lower computational cost and latency than the state-of-the-art methods. In our current implementation, we compress 3D position-based mocap data defined on a skeleton graph. However, it is straightforward to apply our methods to other types of mocap data, such as facial expressions, hand gestures and motion of human bodies. In the future, we will extend our methods to compress mocap data represented by Euler angles. Due to the nonlinear nature of angles, the hierarchical structure may produce significant accumulation errors in the compressed data (Arikan, 2006; Chew et al., 2011). We will seek effective data-driven techniques to tackle this challenge.