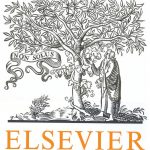
ترجمه مقاله نقش ضروری ارتباطات 6G با چشم انداز صنعت 4.0
- مبلغ: ۸۶,۰۰۰ تومان
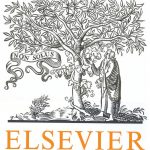
ترجمه مقاله پایداری توسعه شهری، تعدیل ساختار صنعتی و کارایی کاربری زمین
- مبلغ: ۹۱,۰۰۰ تومان
Abstract:
We propose an automatic method for fast reconstruction of indoor scenes from raw point scans, which is a fairly challenging problem due to the restricted accessibility and the cluttered space for indoor environment. We first detect and remove points representing the ground, walls and ceiling from the input data and cluster the remaining points into different groups, referred to as sub-scenes. Our approach abstracts the sub-scenes with geometric primitives, and accordingly constructs the topology graphs with structural attributes based on the functional parts of objects (namely, anchors). To decompose sub-scenes into individual indoor objects, we devise an anchor-guided subgraph matching algorithm which leverages template graphs to partition the graphs into subgraphs (i.e., individual objects), which is capable of handling arbitrarily oriented objects within scenes. Subsequently, we present a data-driven approach to model individual objects, which is particularly formulated as a model instance recognition problem. A Randomized Decision Forest (RDF) is introduced to achieve robust recognition on decomposed indoor objects with raw point data. We further exploit template fitting to generate the geometrically faithful model to the input indoor scene. We visually and quantitatively evaluate the performance of our framework on a variety of synthetic and raw scans, which comprehensively demonstrates the efficiency and robustness of our reconstruction method on raw scanned point clouds, even in the presence of noise and heavy occlusions.
7. Conclusions
We have presented a framework to efficiently decompose and reconstruct indoor scenes directly over raw scanned point clouds. The approach proceeds automatically without user interactions, and thus it is quite appropriate to real-time largescale scanning, modeling and understanding applications. By introducing the anchor-guided strategy, our modeling method is capable of dealing with randomly arranged objects within complex, cluttered indoor scenes, instead of assuming all objects are always oriented with the upward direction. Based on the topology graphs of objects, our graph matching method is able to effectively decompose complex, cluttered scenes and detect individual indoor objects successfully. Furthermore, it is robust to noise and outliers by abstracting scenes with primitives. With discriminative feature descriptors defined, our recognition algorithm is able to tolerate a reasonably high level of data noise, outliers and sparsity. A variety of experiments on raw scans have demonstrated that our reconstruction method can generally produce geometrically faithful results from indoor scenes, even in the presence of severe data imperfection. As discussed, we consider as the anchors the functional parts of indoor objects and our modeling method proceeds with the anchors guided. In case the anchors are missing from primitive fitting, we may not be able to detect the associated objects and consequently the reconstructed scenes are incomplete. Therefore, to seek more robust way to detect anchors needs to be studied in the future work.