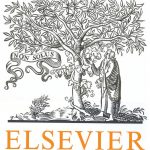
ترجمه مقاله نقش ضروری ارتباطات 6G با چشم انداز صنعت 4.0
- مبلغ: ۸۶,۰۰۰ تومان
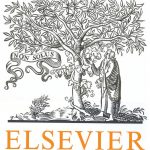
ترجمه مقاله پایداری توسعه شهری، تعدیل ساختار صنعتی و کارایی کاربری زمین
- مبلغ: ۹۱,۰۰۰ تومان
abstract
Constrained approaches to maximum likelihood estimation in the context of finite mixtures of normals have been presented in the literature. A fully data-dependent soft constrained method for maximum likelihood estimation of clusterwise linear regression is proposed, which extends previous work in equivariant data-driven estimation of finite mixtures of normals. The method imposes soft scale bounds based on the homoscedastic variance and a cross-validated tuning parameter c. In our simulation studies and real data examples we show that the selected c will produce an output model with clusterwise linear regressions and clustering as a most-suited-to-the-data solution in between the homoscedastic and the heteroscedastic models.
6. Conclusions
In the present paper, a scale equivariant soft constrained approach to maximum likelihood estimation of clusterwise linear regression model is formulated. This extends the approach proposed in RGD (2017) for multivariate mixtures of normals to the clusterwise linear regression context. Through the extensive simulation studies and the four empirical applications, we are able to show that the method does not only solve the issue of unboundedness, but it is also able to improve upon the unconstrained approaches it was compared with. Whenever either of the unconstrained approaches is instead optimal, our constrained estimator keeps up closely. In addition, as pointed out by the empirical examples, the BIC based on the constrained solution is able to provide sensible choices for the number of clusters whereby the two unconstrained competitors cannot. Whereas RGD (2017) showed that the method has merit in both fuzzy and crisp classification, the additional step ahead we take is twofold: 1) we show that the method works well also when conditional distributions (linear regressions) are specified for the mixture components, by looking at cluster recovery and by 2) bringing into focus, in our simulation study, bias and variance of the model parameter estimators, which are both entailed in the mean squared error. Previous work on constrained estimation of switching regressions [20,30] had shown consistency of the estimator for fixed c, and for c approaching zero with sample size going to infinity. We solve the finite sample problem by using a soft approach, which imposes imprecise bounds based on a cross-validated choice of c. The selected c will produce an output model with clusterwise linear regressions and clustering as a most-suited-to-the-data solution in between the homoscedastic and the heteroscedastic models.