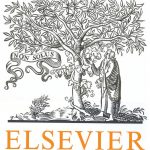
ترجمه مقاله نقش ضروری ارتباطات 6G با چشم انداز صنعت 4.0
- مبلغ: ۸۶,۰۰۰ تومان
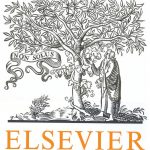
ترجمه مقاله پایداری توسعه شهری، تعدیل ساختار صنعتی و کارایی کاربری زمین
- مبلغ: ۹۱,۰۰۰ تومان
Abstract
The support vector machine (SVM) is a state-of-the-art method in supervised classification. In this paper the Cluster Support Vector Machine (CLSVM) methodology is proposed with the aim to increase the sparsity of the SVM classifier in the presence of categorical features, leading to a gain in interpretability. The CLSVM methodology clusters categories and builds the SVM classifier in the clustered feature space. Four strategies for building the CLSVM classifier are presented based on solving: the SVM formulation in the original feature space, a quadratically constrained quadratic programming formulation, and a mixed integer quadratic programming formulation as well as its continuous relaxation. The computational study illustrates the performance of the CLSVM classifier using two clusters. In the tested datasets our methodology achieves comparable accuracy to that of the SVM in the original feature space, with a dramatic increase in sparsity.
5. Conclusions
In this paper the CLSVM methodology is proposed, based on the SVM with the linear kernel and performing a clustering for categorical features and building an SVM classifier in the clustered feature space. Four strategies are presented to build the CLSVM classifier by means of QCQP, MIQP and QP formulations. When using two clusters, the CLSVM classifier has a comparable classi- fication accuracy to the SVMO classifier, in nine of the twelve benchmark datasets. In the remaining three datasets, the CLSVM classifier outperforms the SVMO classifier in two datasets, and is outperformed in the other one. In terms of sparsity of the classifier with respect to the categorical features, the CLSVM methodology shows a dramatic improvement over the SVMO.