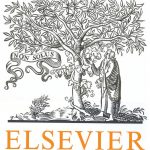
ترجمه مقاله نقش ضروری ارتباطات 6G با چشم انداز صنعت 4.0
- مبلغ: ۸۶,۰۰۰ تومان
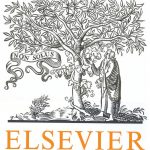
ترجمه مقاله پایداری توسعه شهری، تعدیل ساختار صنعتی و کارایی کاربری زمین
- مبلغ: ۹۱,۰۰۰ تومان
abstract
Clustering analysis has been applied in all aspects of data mining. Density-based and grid-based clustering algorithms are used to form clusters from the core points or dense grids to extend to the boundary of the clusters. However, deficiencies are still existed. To find out the right boundary and improve the precision of the cluster, this paper has proposed a new clustering algorithm (named C-USB) based on the skew characteristic of the data distribution in the cluster margin region. The boundary degree calculated by skew degree and the local density are used to distinguish whether a data is an internal point or non-internal point. And the connected matrix is constructed by removing the neighbor relationships of non-internal points from the relationships of all points, then the clusters can be formed by searching from the connected matrix towards internal of the clusters. Experimental results on synthetic and real data sets show that the C-USB has higher accuracy than that of similar algorithms.
5. Conclusion
Based on the skewed distribution of data points in the boundary area, this paper has proposed a skewness-based measurement method. This method can effectively measure the boundary degree of data points and distinguish the boundary points accurately of the data. The performance of clustering or is on par with the existing latest algorithms. In addition, the boundary detected by the algorithm stated in the paper can better represent the actual situation of clustering boundaries, which playing significant role in data mining.