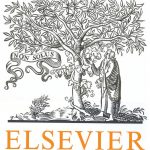
ترجمه مقاله نقش ضروری ارتباطات 6G با چشم انداز صنعت 4.0
- مبلغ: ۸۶,۰۰۰ تومان
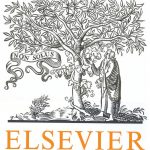
ترجمه مقاله پایداری توسعه شهری، تعدیل ساختار صنعتی و کارایی کاربری زمین
- مبلغ: ۹۱,۰۰۰ تومان
abstract
Classification images have become popular tools in psychophysics, yet difficulties associated with their interpretation have often hindered their application. Alternative methods for characterizing perceptual filters have been proposed, and the discussion has often focussed on the degree to which classification images are optimal statistical estimators of system components (e.g. kernels). This technical note argues that those difficulties become irrelevant once the tool is situated within a data-driven interpretational framework. Within this framework, classification images and their nonlinear derivatives are understood not as transparent estimates of system components, but instead as transparent descriptors of data structure. The many pitfalls associated with the former approach, and the power of the latter, are demonstrated via combination of counter-intuitive computer simulations with empirical examples from published literature. A change in perspective over the manner in which this tool is understood and utilized may lead to a more productive engagement with this methodology.
Final remarks
During the past two decades since the introduction of classification images into vision (Ahumada, 1996), very substantial progress has been made in understanding their relationship to different theoretical and computational constructs (Murray, 2011; Neri, 2010b). This valuable body of knowledge is there to stay, largely unchallenged by the considerations made here. At the same time, an important lesson we have learnt from classification images is that they are most useful when approached as rich descriptions of data structure, rather than transparent images of underlying perceptual mechanisms. We have offered a specific example of how this approach may avoid potentially incorrect interpretations of measured changes in CI structure (Taylor et al., 2014) (Fig. 6). Below we discuss two relevant examples from the broader CI literature where this approach has produced useful developments. An example of interest is the refined understanding of the oftenmeasured differences between target-present and target-absent CI’s (Abbey & Eckstein, 2006; Ahumada et al., 1975; Neri & Heeger, 2002; Solomon, 2002). It was realized in the early stages of psychophysical research on classified noise that those results present significant challenges for the interpretation of CI’s, and that the observed differences most likely reflect the operation of a MAX-like operator connected with perceptual uncertainty (Ahumada et al., 1975). However, the underlying theoretical framework was not formalized until more recently, and in particular with two developments in the 2000’s: an explicit analysis of the connection between uncertainty models and CI’s on the one hand (Tjan & Nandy, 2006), and a general formulation of the connection between nonlinear operators and CI’s on the other hand (Neri, 2004). When combined within a unified framework, these two developments lead to a formal theory of the expected differences between target-present and target-absent CI’s (Neri, 2010c).