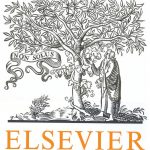
ترجمه مقاله نقش ضروری ارتباطات 6G با چشم انداز صنعت 4.0
- مبلغ: ۸۶,۰۰۰ تومان
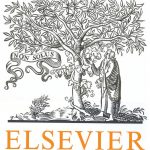
ترجمه مقاله پایداری توسعه شهری، تعدیل ساختار صنعتی و کارایی کاربری زمین
- مبلغ: ۹۱,۰۰۰ تومان
Abstract
In the United States, advances in technology and medical sciences continue to improve the general well-being of the population. With this continued progress, programs such as Medicare are needed to help manage the high costs associated with quality healthcare. Unfortunately, there are individuals who commit fraud for nefarious reasons and personal gain, limiting Medicare’s ability to efectively provide for the healthcare needs of the elderly and other qualifying people. To minimize fraudulent activities, the Centers for Medicare and Medicaid Services (CMS) released a number of “Big Data” datasets for diferent parts of the Medicare program. In this paper, we focus on the detection of Medicare fraud using the following CMS datasets: (1) Medicare Provider Utilization and Payment Data: Physician and Other Supplier (Part B), (2) Medicare Provider Utilization and Payment Data: Part D Prescriber (Part D), and (3) Medicare Provider Utilization and Payment Data: Referring Durable Medical Equipment, Prosthetics, Orthotics and Supplies (DMEPOS). Additionally, we create a fourth dataset which is a combination of the three primary datasets. We discuss data processing for all four datasets and the mapping of real-world provider fraud labels using the List of Excluded Individuals and Entities (LEIE) from the Ofce of the Inspector General. Our exploratory analysis on Medicare fraud detection involves building and assessing three learners on each dataset. Based on the Area under the Receiver Operating Characteristic (ROC) Curve performance metric, our results show that the Combined dataset with the Logistic Regression (LR) learner yielded the best overall score at 0.816, closely followed by the Part B dataset with LR at 0.805. Overall, the Combined and Part B datasets produced the best fraud detection performance with no statistical diference between these datasets, over all the learners. Therefore, based on our results and the assumption that there is no way to know within which part of Medicare a physician will commit fraud, we suggest using the Combined dataset for detecting fraudulent behavior when a physician has submitted payments through any or all Medicare parts evaluated in our study.
Conclusion
Te importance of reducing Medicare fraud, in particular for individuals 65 and older, is paramount in the United States as the elderly population continues to grow. Medicare is necessary for many citizens, and therefore, the importance placed on quality research into fraud detection to keep healthcare costs fair and reasonable. CMS has made available several Big Data Medicare claims datasets for public use over an ever-increasing number of years. Troughout this work, we provide a unique approach (combining multiple Medicare datasets and leverage state-of-the-art Big Data processing and machine learning approaches) for determining the fraud detection capabilities of three Medicare datasets, individually and combined, using three learners, against real-world fraudulent physicians and other medical providers taken from the LEIE dataset. We present our methods for processing each dataset from CMS, the Combined dataset, as well as the mapping of provider fraud labels. We ran experiments on all four datasets: Part B, Part D, DMEPOS, and Combined. Each dataset was considered Big Data, requiring us to employ Spark on top of a Hadoop YARN cluster for running and validating our models. Each dataset was trained and evaluated using three learners: Random Forest, Gradient Boosted Trees and Logistic Regression. Te Combined dataset had the best overall fraud detection performance with an AUC of 0.816 using LR, indicating better performance than each of its individual Medicare parts, and scored similarly to Part B with no signifcant diference in average AUC. Te DMEPOS dataset had the lowest overall results for all learners. Terefore, from these experimental fndings and observations, coupled with the notion that a physician/provider can commit fraud using any part of Medicare, we show that using the Combined dataset with LR provides the best overall fraud detection performance. Future work will include employing data sampling techniques to combat the imbalanced nature of known fraud events in evaluating the diferent Medicare datasets.