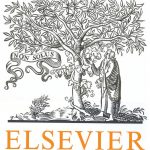
ترجمه مقاله نقش ضروری ارتباطات 6G با چشم انداز صنعت 4.0
- مبلغ: ۸۶,۰۰۰ تومان
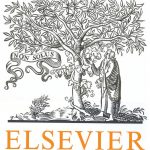
ترجمه مقاله پایداری توسعه شهری، تعدیل ساختار صنعتی و کارایی کاربری زمین
- مبلغ: ۹۱,۰۰۰ تومان
Abstract
Deep Learning and Big Data analytics are two focal points of data science. Deep Learning models have achieved remarkable results in speech recognition and computer vision in recent years. Big Data is important for organizations that need to collect a huge amount of data like a social network and one of the greatest assets to use Deep Learning is analyzing a massive amount of data (Big Data). This advantage makes Deep Learning as a valuable tool for Big Data. Deep Learning can be used to extract incredible information that buried in a Big Data. The modern stock market is an example of these social networks. They are a popular place to increase wealth and generate income, but the fundamental problem of when to buy or sell shares, or which stocks to buy has not been solved. It is very common among investors to have professional fnancial advisors, but what is the best resource to support the decisions these people make? Investment banks such as Goldman Sachs, Lehman Brothers, and Salomon Brothers dominated the world of fnancial advice for more than a decade. However, via the popularity of the Internet and fnancial social networks such as StockTwits and SeekingAlpha, investors around the world have new opportunity to gather and share their experiences. Individual experts can predict the movement of the stock market in fnancial social networks with the reasonable accuracy, but what is the sentiment of a mass group of these expert authors towards various stocks? In this paper, we seek to determine if Deep Learning models can be adapted to improve the performance of sentiment analysis for StockTwits. We applied several neural network models such as long short-term memory, doc2vec, and convolutional neural networks, to stock market opinions posted in StockTwits. Our results show that Deep Learning model can be used efectively for fnancial sentiment analysis and a convolutional neural network is the best model to predict sentiment of authors in StockTwits dataset.
Conclusions
Deep Learning has good performance and promise in many areas, such as natural language processing. Deep Learning has this opportunity to address the data analysis and learning problems in Big Data. In contrast to data mining approaches with its shallow learning process, Deep Learning algorithms transform inputs through more layers. Hidden layers in Deep Learning are generally used to extract features or data representations. Tis hierarchical learning process in Deep Learning provides the opportunity to fnd word semantics and relations. Tese attributes make Deep Learning one of the most desirable models for sentiment analysis.
In this paper, based on our results we show that convolutional neural networks can overcome data mining approach in stock sentiment analysis. In standard data mining approach to text categorization, documents represent as bag-of-word vectors. Tese vectors represent which words appear in a document but do not consider the order of the words in a sentence. It is clear that in some cases, the word order can change the sentiment of a sentence. One remedy to this problem is using bi-grams or n-gram in addition to uni-gram [86, 105, 106]. Unfortunately, using n-grams with n > 1 is not efective [107]. Using CNN provides this opportunity to use n-grams to extract the sentiment of a document efectively. It benefts from the internal structure of data that exists in a document through convolution layers, where each computation unit responds to a small region of input data. We used logistic regression, which works based on a bagof-words, as a baseline and compared the result of applying Deep Learning to logistic regression. Based on our results, among diferent common Deep Learning methods in sentiment analysis, only convolutional neural network outperforms logistic regression. Te accuracy of convolutional neural networks, in comparison to the other models, is considerably better. Based on our results we can use CNN to extract the sentiment of authors regarding stocks from their words. Tere are some people in the fnancial social network who can correctly predict the stock market. By using CNN to predict their sentiment we can predict future market movement.