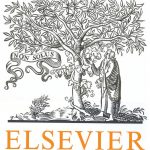
ترجمه مقاله نقش ضروری ارتباطات 6G با چشم انداز صنعت 4.0
- مبلغ: ۸۶,۰۰۰ تومان
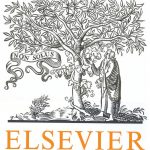
ترجمه مقاله پایداری توسعه شهری، تعدیل ساختار صنعتی و کارایی کاربری زمین
- مبلغ: ۹۱,۰۰۰ تومان
ABSTRACT
The digitalization of the healthcare system has resulted in a deluge of clinical Big Data and has prompted the rapid growth of data science in medicine. Data science, which is the field of study dedicated to the principled extraction of knowledge from complex data, is particularly relevant in the critical care setting. The availability of large amounts of data in the intensive care unit, the need for better evidence-based care, and the complexity of critical illness makes the use of data science techniques and data-driven research particularly appealing to intensivists. Despite the increasing number of studies and publications in the field, so far there have been few examples of data science projects that have resulted in successful implementations of data-driven systems in the intensive care unit. However, given the expected growth in the field, intensivists should be familiar with the opportunities and challenges of Big Data and data science. In this paper, we review the definitions, types of algorithms, applications, challenges, and future of Big Data and data science in critical care.
Clustering and phenotyping
Unsupervised learning algorithms in critical care have mainly been used to uncover naturally occurring subgroups or clusters of patients who share similar clinical and/or molecular characteristics. These clusters are oftentimes called phenotypes, subphenotypes, or subtypes, although there is still little consensus on the terminology.16 For example, Calfee and colleagues applied Latent Class Analysis and identified two subphenotypes of acute respiratory distress syndrome (ARDS) using clinical and cytokine data from two ARDS randomized controlled trials.37 The subphenotypes identified had distinct differences in inflammatory profiles, response to ventilator strategies, and clinical outcomes. Knox and colleagues used selforganizing maps and k-means clustering to identify four distinct clusters of patients with sepsisassociated multiple organ dysfunction syndrome that were independently associated with outcomes after adjusting for severity of illness.38 Luo and colleagues analyzed multiple physiological variable trends of patients in the MIMIC dataset and applied non-negative matrix factorization to group related trends, which were shown to effectively predict 30-day mortality while maintaining model interpretability.39 Finally, Vranas and colleagues applied clustering analysis to discover and validate six clinically recognizable subgroups of ICU patients that differed significantly in all baseline characteristics and clinical trajectories despite sharing common diagnoses.