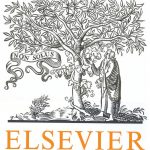
ترجمه مقاله نقش ضروری ارتباطات 6G با چشم انداز صنعت 4.0
- مبلغ: ۸۶,۰۰۰ تومان
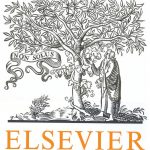
ترجمه مقاله پایداری توسعه شهری، تعدیل ساختار صنعتی و کارایی کاربری زمین
- مبلغ: ۹۱,۰۰۰ تومان
Abstract
A statistical approach to abstract and predict turbine states in an online manner has been developed. Online inference is performed on temperature measurement residuals to predict the failure state ∆n steps ahead of time. In this framework a case study is performed showing the ability to predict bearing failure 33 days, on average, ahead of time. The approach is based on the separability of the sufficient statistics and a hidden variable, namely the state length. The predictive probability is conditioned on the data available, as well as the state variables. It is shown that the predictive probability can be calculated by a model for the samples and a hazard function describing the probability for undergoing a state transition. This study is concerned with the prior training of the model, for which run-to-failure time series of bearing measurements are used. For the sample model prediction is conditioned on prior information and predict the next ∆n samples from a feature space spanned by the prior samples. By assuming that the feature space can be described by a multivariate Gaussian distribution, the prediction is treated as a Gaussian process over the feature space.
6. Conclusion
A state prediction approach has been presented based on the inference of wind turbine bearing temperature residuals and Gaussian processes. Including event data from the individual turbines, it has been shown that prediction of a selected failure event, namely Bearing Over-temperature, is possible. Although evaluated on a limited set of time series, the approach has shown promising results, with an averaged time of prediction a month before the actual time of failure with high confidence, and an accuracy and precision in the order of days and a week, respectively.
For the three time series under consideration, it was noticed that only one out of three predictions converges to the true time of the fault. The others, though close, do not fully converge, with one even showing lower confidence on the partial convergence. Without including more time series, it is not possible to draw certain conclusions. However, it is believed that the discrepancy in the performance can be attributed to the strong data driven nature of the model and the underlying training data. The need to specify the hyper-parameters across all possible states, while training, is a task that requires numerical solutions for the global extrema of highly non-convex cost functions. As such, the calculations become more time consuming when searching for the true optimum, so the model construction becomes a trade-off between computational efficiency and overall model performance.
Exploring the ability of Bayesian inference to abstract and predict wind turbine states conditioned on specific events in more general cases, e.g. using multiple sensors inputs rather than residuals, is a great opportunity for further research.