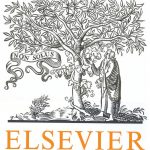
ترجمه مقاله نقش ضروری ارتباطات 6G با چشم انداز صنعت 4.0
- مبلغ: ۸۶,۰۰۰ تومان
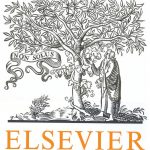
ترجمه مقاله پایداری توسعه شهری، تعدیل ساختار صنعتی و کارایی کاربری زمین
- مبلغ: ۹۱,۰۰۰ تومان
Abstract
In this paper, we tackle the Wireless Body area Network (WBAN) handover issue where a mobile patient has to select at any time the best access technology according to multiple criteria. We particularly focus on the decision schemes and investigate the Multi-Attribute Decision Making (MADM) methods. The fundamental objective of the MADM methods is to determine among a finite set of alternatives the optimal one. Therefore, we propose a Multi-Attribute Decision Making Handover Algorithm (MADMHA) which helps patient’s mobile terminal to dynamically select the best network by providing a ranking order between the list of available candidates. It is a seamless handover approach that guarantees continuous connectivity with respect to the QoS requirements of the WBAN generated traffic types, network history and user preference. Simulation results prove the efficiency of our proposed approach versus the Received Signal Strength Indicator (RSSI) and Data Rate (DR) based handover approaches. Indeed, compared to these latters, MADMHA significantly reduces the packet overhead and the number of handover, while limiting the packet loss ratio.
7. Conclusion and future works
In this paper we addressed the handover issue in Wireless Body Area Networks, focusing our attention on three radio technologies (namely WiFi, 3G and 4G access networks). In particular, we proposed a hybrid Decision Making Handover Algorithm, named MADMHA, which ensures ubiquitous connectivity for mobile WBAN users throughout their roaming. Furthermore, MADMHA classifies the generated healthcare traffic into three classes having heterogeneous QoS requirements (General Monitoring, Delay Sensitive and Mandatory Emergency). Based on such classification, MADMHA performs an intelligent network selection according to QoS requirements of each application type, while further taking into account the network history. We performed a thorough numerical analysis of the proposed algorithm, showing that our approach achieves very good results in terms of number of handover experienced by users, energy consumption, packet overhead and reliability. Three interesting areas for future work can be identified in the context of the presented work. MADMHA solution that we develop allows us to consider additional attributes and to define various relative importance between such attributes. Indeed, other network attributes (e.g., security of the candidate network) can be added to consider more selection objectives. Another issue concerns the setting of weighting parameters. How can we define exactly the relative importance of attributes? In our context, the weights are estimated by human judgement. However, the scale of relative importance between attributes can be chosen by using an autolearning algorithm. Besides, the network performance in terms of algorithm complexity and convergence speed may be addressed in our future works and validated through real test scenarios.