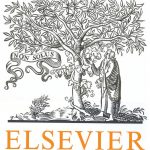
ترجمه مقاله نقش ضروری ارتباطات 6G با چشم انداز صنعت 4.0
- مبلغ: ۸۶,۰۰۰ تومان
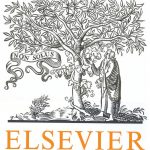
ترجمه مقاله پایداری توسعه شهری، تعدیل ساختار صنعتی و کارایی کاربری زمین
- مبلغ: ۹۱,۰۰۰ تومان
Abstract
Vehicle tracking task plays an important role on the Internet of vehicles and intelligent transportation system. Beyond the traditional Global Positioning System sensor, the image sensor can capture different kinds of vehicles, analyze their driving situation, and can interact with them. Aiming at the problem that the traditional convolutional neural network is vulnerable to background interference, this article proposes vehicle tracking method based on human attention mechanism for self-selection of deep features with an inter-channel fully connected layer. It mainly includes the following contents: (1) a fully convolutional neural network fused attention mechanism with the selection of the deep features for convolution; (2) a separation method for template and semantic background region to separate target vehicles from the background in the initial frame adaptively; (3) a two-stage method for model training using our traffic dataset. The experimental results show that the proposed method improves the tracking accuracy without an increase in tracking time. Meanwhile, it strengthens the robustness of algorithm under the condition of the complex background region. The success rate of the proposed method in overall traffic datasets is higher than Siamese network by about 10%, and the overall precision is higher than Siamese network by 8%.
Conclusion and future work
In this article, we focus on the vehicle tracking task for IOV and propose the SAS-Net model which combines the bilinear network with a visual attention mechanism, making the model select the features from different channels according to different impacts of features from semantic areas. The model makes the vehicle no longer disturbed by the background area and the occluded vehicles and has obtained good results compared with other methods. The success rate of SAS-Net tracking is higher than that of KCF, DSST, and Siamese-FC under most overlapping rate thresholds. The precision of SAS-Net is much higher than the other three methods when the distance threshold is below 30. Our SAS-Net achieves a real-time tracking speed. If there is more hardware support, this model can be applied to largescale intelligent IOV system to assist all the connected vehicles to make a good travel plan. In the future research work, the joint vehicle tracking method based on the multi-image sensor will be brilliant. In the IOV system, interaction and fusion of information generated by different sensors among different vehicles are inevitable. In the combination of multi-sensors, how to fuse the multimodal data from various kinds of sensors, such as image sensors and wireless sensors, will be a long-term research goal. Deep neural networks still have great potential in these fields. Designing different kinds of neural networks for these multimodal data can achieve the fusion of different sensor information.