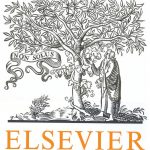
ترجمه مقاله نقش ضروری ارتباطات 6G با چشم انداز صنعت 4.0
- مبلغ: ۸۶,۰۰۰ تومان
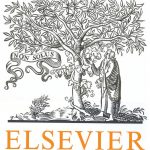
ترجمه مقاله پایداری توسعه شهری، تعدیل ساختار صنعتی و کارایی کاربری زمین
- مبلغ: ۹۱,۰۰۰ تومان
Abstract
Rapid advances in hardware-based technologies during the past decades have opened up new possibilities for life scientists to gather multimodal data in various application domains, such as omics, bioimaging, medical imaging, and (brain/body)–machine interfaces. These have generated novel opportunities for development of dedicated data-intensive machine learning techniques. In particular, recent research in deep learning (DL), reinforcement learning (RL), and their combination (deep RL) promise to revolutionize the future of artificial intelligence. The growth in computational power accompanied by faster and increased data storage, and declining computing costs have already allowed scientists in various fields to apply these techniques on data sets that were previously intractable owing to their size and complexity. This paper provides a comprehensive survey on the application of DL, RL, and deep RL techniques in mining biological data. In addition, we compare the performances of DL techniques when applied to different data sets across various application domains. Finally, we outline open issues in this challenging research area and discuss future development perspectives.
CONCLUSION
The recent bliss of technological advancement in life sciences came with the huge challenge of mining the multimodal, multidimentional, and complex biological data. Triggered by that call, interdisciplinary approaches have resulted in the development of cutting edge machine learning-based analytical tools. The success stories of ANN, deep architectures, and reinforcement learning in making machines more intelligent are well known. Furthermore, computational costs have dropped, computing power has surged, and quasi-unlimited solid-state storage is available at a reasonable price. These factors have allowed to combine these learning techniques to reshape machines’ capabilities to understand and decipher complex patterns from biological data. To facilitate wider deployment of such techniques and to serve as a reference point for the community, this paper provides a comprehensive survey of the literature of techniques’ usability with different biological data. Further, a comparative study is presented on performances of various DL techniques, when applied to data from different biological application domains, as reported in the literature. Finally, some open issues and future perspectives are highlighted.