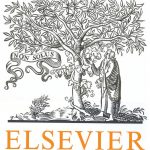
ترجمه مقاله نقش ضروری ارتباطات 6G با چشم انداز صنعت 4.0
- مبلغ: ۸۶,۰۰۰ تومان
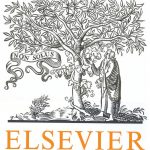
ترجمه مقاله پایداری توسعه شهری، تعدیل ساختار صنعتی و کارایی کاربری زمین
- مبلغ: ۹۱,۰۰۰ تومان
Abstract:
The quality of a dataset plays a central role in the results and conclusion that can be drawn from analysis such a dataset. As it is often said; garbage in, garbage out. In recently years, neural networks have displayed good performance in solving a diverse number of problems. Unfortunately, artificial neural networks are not immune to this misfortune presented by missing values. Furthermore, in most real word settings, it is often the case that, the only data available for the training of artificial neural networks consists of a significant amount of missing values. In such cases, we are left with little choice but to use this data for the purposes of training neural networks, although doing so may result in a poorly performing neural network. In this paper, we describe the use of neural network dropout as a technique for training neural networks in the presence of missing values. We test the performance of different neural network architectures on different levels of artificial generated missing values introduces on the MNIST handwriting recognition dataset, Cifar-10 and the Pima Indians Diabetes Dataset and find that in most cases it results in significantly better performance of the neural network compared to other missing data handling techniques.
Conclusions
This paper discussed results observed when training numerous neural network models using ANN-MIND. The results showed that compared to other techniques we looked at, ANNMIND performs well (in terms of accuracy) in the presence of missing values. Furthermore, we saw that in the presence of no missing data, ANN-MIND performs just as well as a similar network without ANN-MIND applied. The model we proposed in this paper showed impressive performance in the cases where the data at our disposal was missing. The model showed impressive performance on both the MNIST dataset (in which we artificially created missing data) and the mushroom edibility classification dataset (which has missing values in its natural state and as such didn’t require any artificial data manipulation). Over the cause of conducting this research study, the author found that, whilst the presence of missing data is a norm in industry, finding a public dataset that has a lot of missing values is a time-consuming process. The absence of a standardised dataset with which any proposed missing data model’s efficiency could be verified against means that measuring the efficiency of a model proposed by other researchers is extremely difficult.