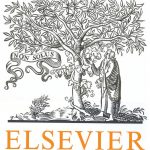
ترجمه مقاله نقش ضروری ارتباطات 6G با چشم انداز صنعت 4.0
- مبلغ: ۸۶,۰۰۰ تومان
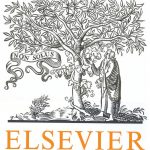
ترجمه مقاله پایداری توسعه شهری، تعدیل ساختار صنعتی و کارایی کاربری زمین
- مبلغ: ۹۱,۰۰۰ تومان
Abstract
Decentralized energy supply systems (DESS) are highly integrated and complex systems designed to meet time-varying energy demands, e.g., heating, cooling, and electricity. The synthesis problem of DESS addresses combining various types of energy conversion units, choosing their sizing and operations to maximize an objective function, e.g., the net present value. In practice, investment costs and part-load performances are nonlinear. Thus, this optimization problem can be modeled as a nonconvex mixed-integer nonlinear programming (MINLP) problem. We present an adaptive discretization algorithm to solve such synthesis problems containing an iterative interaction between mixed-integer linear programs (MIPs) and nonlinear programs (NLPs). The proposed algorithm outperforms state-of-the-art MINLP solvers as well as linearization approaches with regard to solution quality and computation times on a test set obtained from real industrial data, which we made available online.
5. Summary and outlook
The superstructure-based synthesis of decentralized energy supply systems can be formulated as a mixed-integer nonlinear program. By including, e.g., nonlinear part-load performances, investment costs, and strict energy balances, this optimization problemis unavoidablynonconvex.Inthis paper, we donot circumvent this issue via approximating the problem by linearizing the performance and investment cost models of all considered types of equipment. Such approximate solutions from linearized problems are not necessarily feasible for the original nonlinear problem. In this paper, we setthe focus on computing solutions which are feasible for the nonlinear synthesis problem. Since global MINLP solvers, e.g., BARON, have difficulties to provide primal solutions for realworld-based testinstances, we propose a problem-specific solution approach for the nonlinear synthesis of DESS. This optimizationbased algorithm consists of a discretized and linearly formulated version of the synthesis problem, whose underlying discretization grid is iteratively adapted. The resulting approximate problem is of such a nature that solution-specific decisions are also feasible in the original nonlinear problem and by solving a decomposable NLP, this leads to MINLP solutions. A computational study based on a set of test instances obtained from real industrial data shows that the proposed adaptive discretization algorithm computes better MINLP solutions in less computation times than state-ofthe-art solvers. Thus, the proposed algorithm provides an efficient solution method to the synthesis of decentralized energy supply systems. In future work, one should expand the adaptive discretization algorithm concerning methods to compute dual bounds for the MINLP to estimate the optimality gap of the algorithm’s primal solutions. The (mostly very weak) dual bounds provided by BARON do not contribute meaningful information. A mathematically feasible or even optimal solution is usually only an approximation of a real-world implementation, since a model never represents the real problem perfectly. Real world decisions might be influenced by constraints not represented in the model, e.g., (missing) maintenance knowledge in the company for specific technologies. In Voll et al. (2013a) and Hennen et al. (2016) we show that several near-optimal solution alternatives exist. Analyzing these near-optimal solutions allows to derive real-world decision options. Since the proposed AdaptDiscAlgo efficiently provides feasible solutions of the nonlinear synthesis problem, in future work the algorithmcouldbe expandedto efficiently generate many near-optimal solution alternatives. To identify the suitable superstructure, one could complement the proposed algorithm with the successive superstructure expansion method of Voll et al. (2013b).