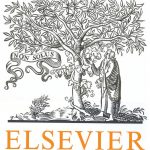
ترجمه مقاله نقش ضروری ارتباطات 6G با چشم انداز صنعت 4.0
- مبلغ: ۸۶,۰۰۰ تومان
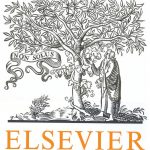
ترجمه مقاله پایداری توسعه شهری، تعدیل ساختار صنعتی و کارایی کاربری زمین
- مبلغ: ۹۱,۰۰۰ تومان
Abstract
Cuckoo optimization algorithm (COA) is inspired from the special and exotic lifestyle of a bird family called the cuckoo and her amazing and unique behavior in egg laying and breeding. Just like any other population-based swarm intelligence metaheuristic algorithms, the basic COA starts with a set of randomly generated solutions called “habitats.” Actually, the habitats can be the current locations of either the mature cuckoos or their eggs. In an iterative manner, cuckoos lay their eggs around their habitats inside the other birds’ nests by mimicking their eggs’ color, pattern, and size, and this is a kind of parasitic brooding behavior. Some hosts may discriminate the strange eggs and throw them out while the others not. The survival competition between cuckoos and their hosts, and migration of cuckoos in swarm are two main underlying motivations to introduce the COA. In this paper, an adaptive cuckoo optimization algorithm named A-COA is proposed in which three novelties in egg-laying and migration phases are applied. These modifications have made the basic algorithm more efficient with faster convergence to solve continuous and discrete optimization problems. A comprehensive comparison study of A-COA versus not only the basic COA but also some other conventional metaheuristics like GA, PSO, ABC, and TLBO has been made on a variety of unimodal and multimodal numerical benchmark functions with different characteristics, and the results show an overall 25.85% of improvement in terms of performance with a faster convergence speed compared to the basic COA, where the statistical Wilcoxon rank-sum test certifies our conclusions. In addition, a discretized version of A-COA and its application to the multiprocessor task scheduling problem as a complex combinatorial optimization problem are investigated where the proposed A-COA is very competitive with not only the strongest conventional heuristics, for example, MCP, ETF, and DLS, but also the basic COA and the newly proposed ACO-based approach.
1 Introduction
Most of scientific, engineering and industrial problems can be formulated as a corresponding continuous or discrete objective function with some global optima and a large number of local minima/maxima around. Actually, such kinds of problems are NP-hard from the time complexity perspective, so that there are no exact algorithms to solve them in polynomial time budget. On this basis, using exact methods is impractical specially where the dimensionality of the problem at hand is high, and hence, metaheuristic algorithms, which have a significant potential as general problem solvers, to solve such kinds of problems have been receiving increasing attention in recent years. Such methods, which most of them are inspired from natural phenomena, are capable of finding at least suboptimal solutions in a significantly reduced time budget. Considering different factors, such methods can be discriminated into the different categories. Of them, two important ones introduced in the literature are evolutionary algorithms (EA) and swarm intelligence (SI) ones.
7 Conclusion
In this paper, an adaptive version of cuckoo optimization algorithm named A-COA with three novelties in the egglaying and migration phases was proposed. (1) The egglaying radius (ELR) was nonlinearly reduced over the iterations for faster convergence, inducing a better balance between exploration and exploitation, especially in the last iterations. (2) After egg-laying phase, those eggs laid in the same locations (with an e tolerance) are recognized and killed except one of them. While in the basic COA the epsilon coefficient was constant all over the execution, in the A-COA this coefficient was decreased linearly with the iterations. This idea lets compacter populations in the last iterations help with the exploitation and local search for getting exact final solutions. (3) In contrast to the basic COA in which the motion coefficient is constant during the algorithm execution, in the A-COA, we applied an adoptive motion coefficient for each cuckoo based on the cuckoo’s distance to the global best; i.e., the farer cuckoo would get higher coefficient to move forward. This idea, on the one hand, helped the farer cuckoos get trapped in the local minima having a big jump toward the global best and exiting off the stagnation while helping the convergence speed; on the other hand, it slowed down the speed of the nearer cuckoos for a better investigation around the global best in order to improve the accuracy as well as avoid premature convergence.
الگوریتم بهینه سازی فاخته (COA)، از سبک زندگی جالب و اجتماعی یک خانواده از پرنده ها با نام فاخته و رفتار جالب و منحصر به فرد او در تخم گذاری و پرورش جوجه های خودش، الهام گرفته شده است. درست مانند هر الگوریتم هوشمند ذرات جمعیتی فرا ابتکاری دیگر، COA اولیه با یک مجموعه از راه کار های رندوم با نام «زیست بوم» کار خودش را شروع می کند. در واقع، زیست بوم ها می تواند جایگاه فعلی فاخته های بالغ و یا تخم های آن ها باشد. در حالت تکراری، فاخته ها تخم های خودشان را اطراف زیستگاه خودشان، داخل لانه پرنده های دیگر قرار می دهند و این کار را با تقلید از رنگ، الگو و سایز تخم های پرنده های دیگر انجام میدهد و این یک نوع از رفتار پرورش انگلی ( یعنی پرورش جوجه های یک پرنده در لانه یک پرنده دیگر)می باشد. بعضی از میزبان ها ممکن است متوجه تفاوت تخم های غریبه با تخم های خودشان بشوند و آن ها را بیرون بیندازند اما بعضی از آن ها هم متوجه نمی شوند. رقابت نجات بین فاخته ها و میزبان آن ها، و مهاجرت فاخته ها به صورت دسته جمعی، دو انگیزه اصلی برای معرفی COA هستند. در این مقاله، یک الگوریتم بهینه سازی تطبیقی فاخته با نام A-COA ارائه شده است که در این الگوریتم، سه روش جدید در تخم گذاری و فاز های مهاجرتی مورد استفاده قرار گرفته است. این اصلاحات باعث شده است که الگوریتم اصلی موثرتر باشد و همگرایی سریع تری برای حل مسئله و بهینه سازی گسسته به دست بیاید. یک مطالعه جامع مقایسه ای بر روی الگوریتم A-COA در مقایسه با COA و همچنین دیگر الگوریتم های فرا ابتکاری مانند GA، PSO، ABC و TLBO با استفاده از توابع معیار چند حالتی و تک حالتی مختلف با مشخصه های گوناگون انجام شد و نتایج نشان میدهد که به صورت کلی 25.85% بهبود از نظر عملکرد با همگرایی سریع تر در مقایسه با COA اولیه به دست آمده است ، و در عین حال تست های آماری رتبه بندی – مجموع ویلکاکسون نیز جمع بندی های ما را تایید می کند. به علاوه، یک نسخه گسسته از A-COA و کاربرد آن بر روی مسئله وظایف زمان بندی پردازشگرهای چندگانه به عنوان یک مسئله بهینه سازی ترکیبی پیچیده در این قسمت بررسی شده است که در آن، A-COA پیشنهاد شده نه تنها با قویترین الگوریتم های متداول فرا ابتکاری مانند MCP، ETF، و DLS قابل مقایسه است بلکه نسبت به COA و روش های جدید مبتنی بر ACO نیز عملکرد بسیار خوبی دارد.
1. مقدمه
بیشتر مسئله های علمی، مهندسی و صنعتی را می توان به صورت توابع هدف پیوسته و گسسته با حالت بهینه سراسری و یک مجموعه بزرگ از اکسترموم های محلی، فرمول بندی کرد. در واقع، این نوع مسئله ها، مسئله های سخت NP از دیدگاه پیچیدگی زمانی هستند، در نتیجه هیچ الگوریتم دقیقی برای حل کردن این بودجه های زمانی چند جمله ای وجود ندارد. بر این اساس، استفاده از روش های دقیق به خصوص زمانی که ابعاد مسئله بالا باشد کار عملی و صحیحی نیست و ازین رو، الگوریتم های فرا اکتشافی که پتانسیل بالایی به عنوان حلال مسئله دارند در سالهای اخیر توجه زیادی را به خودشان جلب کرده اند. این روش ها، که بیشتر آنها الهام گرفته از پدیده های طبیعی هستند، می توانند حداقل راه حلهای نزدیک به بهینه را در بودجه های زمانی بسیار کمتر، به دست بیاورند. با در نظر داشتن روش های مختلف، این روش ها را میتوان در دسته بندی های مختلف در نظر گرفت. از میان آنها، دو مورد مهم آن ها که در مقالات مختلف بررسی شده اند، الگوریتم های تکاملی(EA) و الگوریتم های هوش ذرات (SI) هستند.
7. جمع بندی
در این مقاله یک نسخه تطبیقی از روش بهینه سازی فاخته با نام A-COA ارائه شده است که سه ابتکار در فاز های تخم گذاری و مهاجرت پیشنهاد شده است: 1)شعاع تخم گذاری (ELR) که به صورت غیر خطی در هر تکرار کاهش پیدا می کند تا یک همگرایی سریع تر رخ دهد، و در نتیجه باعث می شود که توازن بهتری بین بهره وری و جستجو ایجاد شود به خصوص در تکرار های آخر. 2) بعد از فاز تخم گذاری، تخم های گذاشته شده در بعضی از مکان ها( با تلورانس اپسیلون) شناسایی شده و کشته می شوند به غیر از یکی از آن ها. در حالی که در COA اولیه این ضریب اپسیلون در تمام اجرا ثابت می باشد، در A-COA این ضریب به صورت خطی با هر تکرار کاهش پیدا می کند. این ایده باعث می شود که جمعیت کمتری در تکرار های آخر ایجاد شود که باعث می شود بهره وری بهتر و جستجوی محلی دقیق تری برای رسیدن به راه حل دقیق ایجاد شود. 3) بر خلاف COA اولیه که در آن ضریب های حرکتی در طول اجرای الگوریتم ثابت هستند، در A-COA ما از ضریب حرکت تطبیقی برای هر فاخته مبتنی بر فاصله فاخته تا بهترین راه حل سراسری استفاده می کنیم؛ یعنی هر چه فاخته دورتر باشد می تواند ضریب بیشتری دریافت کند تا به سمت بهترین راه حل، سریع تر حرکت کند. این ایده در طرف دیگر باعث میشود فاخته های که در مینیموم های محلی گیر کرده اند بتوانند از آن خارج شوند و این موضوع باعث افزایش سرعت همگرایی می شود؛ در طرف دیگر، این کار باعث می شود که سرعت فاخته های نزدیک تر کاهش پیدا کنند تا آن ها بتوانند یک جستجوی بهتر حول بهترین جایگاه سراسری داشته باشند تا دقت کار افزایش یافته و همگرایی زودهنگام نیز شکل نگیرد.