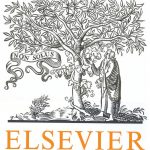
ترجمه مقاله نقش ضروری ارتباطات 6G با چشم انداز صنعت 4.0
- مبلغ: ۸۶,۰۰۰ تومان
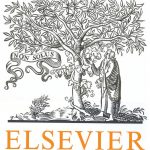
ترجمه مقاله پایداری توسعه شهری، تعدیل ساختار صنعتی و کارایی کاربری زمین
- مبلغ: ۹۱,۰۰۰ تومان
With widespread availability of graph-structured data from sources ranging from social networks to biochemical processes, there is increasing need for efficient and effective graph analyses techniques. Graphs with millions of vertices and beyond are commonplace, necessitating both efficient serial algorithms, as well as scalable parallel formulations. This paper addresses the problem of global graph alignment on supercomputer-class clusters. Given two graphs (or two instances of the same graph), we define graph alignment as a mapping of each vertex in the first graph to a unique vertex in the second graph so as to optimize a given similarity-based cost function1 . Graph alignment is typically implemented in two steps – in the first step, a similarity matrix is computed. Entries in the matrix quantify similarity of node pairs, one chosen from each graph. In the second step, similar vertices are extracted through a bipartite matching algorithm applied to the similarity matrix. Using a state of the art serial algorithm for similarity matrix computation called Network Similarity Decomposition (NSD), we derive corresponding parallel formulations. Coupling this parallel similarity algorithm with a parallel auction-based bipartite matching technique, we derive a complete graph matching pipeline that is highly efficient and scalable. We validate the performance of our integrated approach on a large, supercomputer-class cluster and diverse graph instances (including Protein Interaction (PPI) networks, Web graphs, and Wikipedia link structures). Experimental results demonstrate that our algorithms scale to large machine configurations and problem instances.