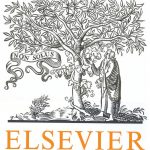
ترجمه مقاله نقش ضروری ارتباطات 6G با چشم انداز صنعت 4.0
- مبلغ: ۸۶,۰۰۰ تومان
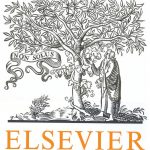
ترجمه مقاله پایداری توسعه شهری، تعدیل ساختار صنعتی و کارایی کاربری زمین
- مبلغ: ۹۱,۰۰۰ تومان
abstract
We investigate the use of Deep Neural Networks for the classification of image datasets where texture features are important for generating class-conditional discriminative representations. To this end, we first derive the size of the feature space for some standard textural features extracted from the input dataset and then use the theory of Vapnik–Chervonenkis dimension to show that hand-crafted feature extraction creates low-dimensional representations which help in reducing the overall excess error rate. As a corollary to this analysis, we derive for the first time upper bounds on the VC dimension of Convolutional Neural Network as well as Dropout and Dropconnect networks and the relation between excess error rate of Dropout and Dropconnect networks. The concept of intrinsic dimension is used to validate the intuition that texture-based datasets are inherently higher dimensional as compared to handwritten digits or other object recognition datasets and hence more difficult to be shattered by neural networks. We then derive the mean distance from the centroid to the nearest and farthest sampling points in an n-dimensional manifold and show that the Relative Contrast of the sample data vanishes as dimensionality of the underlying vector space tends to infinity.
7. Conclusion
The use of Deep Neural Networks for texture recognition has seen a significant impediment due to a lack of thorough understanding of the limitations of existing Neural architectures. In this paper, we provide theoretical bounds on the use of Deep Neural Networks for texture classification. First, using the theory of VC-dimension we establish the relevance of handcrafted feature extraction. As a corollary to this analysis, we derive for the first time upper bounds on the VC dimension of Convolutional Neural Network as well as Dropout and Dropconnect networks and the relation between excess error rates. Then we use the concept of Intrinsic Dimension to show that texture datasets have a higher dimensionality than color/shape based data. Finally, we derive an important result on Relative Contrast that generalizes the one proposed in Aggarwal et al. (2001). From the theoretical and empirical analysis, we conclude that for texture data, we need to redesign neural architectures and devise new learning algorithms that can learn features similar to GLCM and other spatial correlation based texture-features from input data.