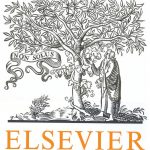
ترجمه مقاله نقش ضروری ارتباطات 6G با چشم انداز صنعت 4.0
- مبلغ: ۸۶,۰۰۰ تومان
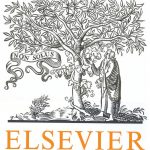
ترجمه مقاله پایداری توسعه شهری، تعدیل ساختار صنعتی و کارایی کاربری زمین
- مبلغ: ۹۱,۰۰۰ تومان
Abstract
Workflows are a set of tasks and the dependency among them, which are divided into scientific and business categories. To avoid problems of centralized execution of workflows, they are broken into segments that is known as fragmentation. To fragment the workflow, it is highly important to consider the dependency among tasks and runtime conditions. The cooperation between the scheduler and fragmentor must be such that the latter generates appropriate tasks with optimized communication cost, delay time, response time, and throughput. To this end, in the present study, a framework is proposed for scheduling and fragmentation of tasks in scientific workflows that are conducted in fragmentation and scheduling phases. In the fragmentation phase, the fragments are generated with regard to the number of virtual machines available during runtime. In the scheduling phase, the virtual machines are selected with the aim of reducing bandwidth usage. The experiments are performed with three Configurations during both phases of fragmentation and scheduling. Response time, throughput, and cost (BW and RAM) were improved compared to the baseline studies on Sipht, Inspiral, Epigenomics, Montage, and CyberShake workflows as datasets.
Conclusions and Future Studies
Workflow fragmentation and scheduling are significant problems in workflow management. Based on the definition by Wei Tan et al., fragmentation is a partition of workflow model. Models proposed for fragmentation have disadvantages such as increased number of generated fragments after fragmentation, which increases communication messages, time delay, and mean response time, thereby reducing throughput. Other disadvantages include static fragment generation which decreases flexibility and efficiency. Thus, the present study proposed a model for fragmentation which dynamically fragmented scientific workflows, considering runtime conditions. Moreover, it resolved the noted problems by controlling fragment generation. In WSADF framework, the number of tasks in each fragment was calculated based on the number of virtual machines. Fragments were generated during the execution, reducing communication messages among the fragments. In this study, WSADF framework was compared with the FPD algorithm in the fragmentation phase, and with FPD, CTC, Centralized, SLV, and QDA algorithms in the scheduling phase. According to the results of the experiments, response time and throughput were improved compared to the baseline studies. As the result of decreasing the number of generated fragments compared to the baseline studies, the number of communication messages among the fragments as well as delay time was reduced in this study, thereby decreasing response time and enhancing throughput. Furthermore, the results of the experiments for bandwidth usage cost and memory cost revealed the improved performance of the proposed framework compared to the baseline studies because the former controlled the number of generated fragments and selected appropriate virtual machines with less cost during runtime. Experiments were conducted in three Configuration and in both phases of fragmentor and scheduler. Results were improved compared to the baseline studies. For instance, compared to Montage workflow in Configuration-1 and the fragmentor phase, it showed 84.75% improvement in mean response time and 87.68% improvement in throughput. In Configuration2 and the fragmentor phase, it showed 84.64% improvement in mean response time and 83.46% improvement in throughput. In Configuration-1 and the scheduler phase, it demonstrated 83.94%, 69.56%, and 96.91% improvement in mean response time, throughput, and bandwidth usage cost, respectively. In Configuration-2 and the scheduler phase, it demonstrated 94.49%, 47.82%, and 96.1% improvement in mean response time, throughput, and bandwidth usage cost, respectively.