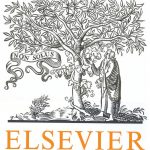
ترجمه مقاله نقش ضروری ارتباطات 6G با چشم انداز صنعت 4.0
- مبلغ: ۸۶,۰۰۰ تومان
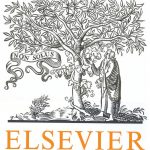
ترجمه مقاله پایداری توسعه شهری، تعدیل ساختار صنعتی و کارایی کاربری زمین
- مبلغ: ۹۱,۰۰۰ تومان
Abstract
Twin Support Vector Machines (TWSVM) is a new machine learning method based on the theory of Support Vector Machine (SVM). Unlike SVM, TWSVM would generate two non-parallel planes, such that each plane is closer to one of the two classes and is as far as possible from the other. In TWSVM, a pair of smaller sized quadratic programming problems (QPPs) is solved, instead of solving a single large one in SVM, making the computational speed of TWSVM approximately 4 times faster than the standard SVM. At present, TWSVM has become one of the popular methods because of its excellent learning performance. In this paper, the research progress of TWSVM is reviewed. Firstly, it analyzes the basic theory of TWSVM, then tracking describes the research progress of TWSVM including the learning model and specific applications in recent years, finally points out the research and development prospects. This helps researchers to effectively use TWSVM as an emerging research approach, encouraging them to work further on performance improvement.
Conclusions and Prospects
At present, TWSVM has become one of the popular methods because of its excellent learning performance. Because TWSVM is a relatively new theory in the field of machine learning, it is not mature and perfect. such as, it is not sparse. At the same time, it has a lower generalization ability. Furthermore, its theory lacks practical application background. Even more, the frame of TWSVM learning theory has yet to be established. Therefore, TWSVM needs further study and improvement. Further research includes: (1) How to construct the perfect kernel function for TWSVM. The kernel function, which is an important part of TWSVM, determines the level of non-linear processing ability. So the kernel function in the TWSVM occupies an extremely important position, which is the key to the mature development of TWSVM theory. So far, there is not yet a clear theoretical result to guide us on how to choose a good performance based on a specific data set kernel function. The selection and construction of kernel functions and the optimization of the corresponding parameters are still an open and urgent problem. (2) How to better solve the sparseness of TWSVM. Lack of sparseness, it will reduce the accuracy of the algorithm. The lack of sparseness is a key problem in the study of TWSVM theory. (3) How to better apply TWSVM to multi-classification. At present, the traditional TWSVM algorithm model is generally applied to two classification problems, how to extend the TWSVM algorithm to multi-classification problem, and achieve the desired computing efficiency, which is worthy of our study. (4) How to extend the scope of TWSVM applications. At present, TWSVM research focused on the theoretical research. How to expand its practical application areas, will be the focus of the next step.