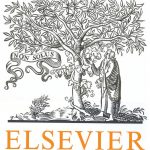
ترجمه مقاله نقش ضروری ارتباطات 6G با چشم انداز صنعت 4.0
- مبلغ: ۸۶,۰۰۰ تومان
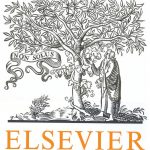
ترجمه مقاله پایداری توسعه شهری، تعدیل ساختار صنعتی و کارایی کاربری زمین
- مبلغ: ۹۱,۰۰۰ تومان
Abstract
In this paper we present a new traffic sign detection and recognition (TSDR) method, which is achieved in three main steps. The first step segments the image based on thresholding of HSI color space components. The second step detects traffic signs by processing the blobs extracted by the first step. The last one performs the recognition of the detected traffic signs. The main contributions of the paper are as follows. First, we propose, in the second step, to use invariant geometric moments to classify shapes instead of machine learning algorithms. Second, inspired by the existing features, new ones have been proposed for the recognition. The histogram of oriented gradients (HOG) features has been extended to the HSI color space and combined with the local self-similarity (LSS) features to get the descriptor we use in our algorithm. As a classifier, random forest and support vector machine (SVM) classifiers have been tested together with the new descriptor. The proposed method has been tested on both the German Traffic Sign Detection and Recognition Benchmark and the Swedish Traffic Signs Data sets. The results obtained are satisfactory when compared to the state-of-the-art methods.
5. Conclusion and perspectives
In this paper, a three stages system for real-time Traffic Sign Detection and Recognition has been presented. The first stage segments the images into ROIs based on color information. Only significant ROIs will be considered referred to their size and aspect ratio constraints. In the second stage, the circular, rectangular and triangular shapes are detected using invariant geometric moments. In the recognition stage, we combine the HOG features computed in the HSI color space with LSS features to form a new descriptor. The Random Forest classifier is used with this descriptor to recognize the detected shapes. The entire system achieves 94.21% AUC on the GTSDB data set at a processing rate of 8–10 frames/s. In the future work, we areplanning touse adaptive thresholding to overcome the color segmentation problems. On the other hand, temporal information could also be integrated to track the detected traffic signs and reinforce the decision making process. This would also allow us to restrict the search space in the current image considering previous detections information, which can accelerate the candidate detection. Moreover, the feature selection can be employed to accelerate the recognition phase by reducing the size ofthe descriptor vectors. Further, this could be combined with other classifiers, such as the Neural Networks.