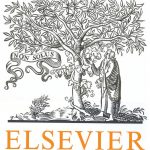
ترجمه مقاله نقش ضروری ارتباطات 6G با چشم انداز صنعت 4.0
- مبلغ: ۸۶,۰۰۰ تومان
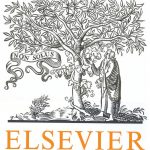
ترجمه مقاله پایداری توسعه شهری، تعدیل ساختار صنعتی و کارایی کاربری زمین
- مبلغ: ۹۱,۰۰۰ تومان
Abstract
Privacy leakage is an important issue for social relationships-based recommender systems (i.e., social recommendation). Existing privacy preserving social recommendation approaches usually allow the recommender to fully control users’ information. This may be problematic since the recommender itself may be untrusted, leading to serious privacy leakage. Besides, building social relationships requires sharing interests as well as other private information, which may lead to more privacy leakage. Although sometimes users are allowed to hide their sensitive private data using personalized privacy settings, the data being shared can still be abused by the adversaries to infer sensitive private information. Supporting social recommendation with least privacy leakage to untrusted recommender and other users (i.e., friends) is an important yet challenging problem. In this paper, we aim to achieve privacy-preserving social recommendation under personalized privacy settings. We propose PrivSR, a novel privacy-preserving social recommendation framework, in which user can model user feedbacks and social relationships privately. Meanwhile, by allocating different noise magnitudes to personalized sensitive and non-sensitive feedbacks, we can protect users’ privacy against untrusted recommender and friends. Theoretical analysis and experimental evaluation on real-world datasets demonstrate that our framework can protect users’ privacy while being able to retain effectiveness of the underlying recommender system.
8 Conclusion and future work
In this paper, we study the problem of privacy-preserving social recommendation with personalized privacy settings. We propose a novel differential privacy-preserving framework in a semi-centralized way which can protect users’ sensitive ratings while being able to retain the effectiveness of recommendation. Theoretic analysis and experimental evaluation on real-world datasets demonstrate the effectiveness of the proposed framework for recommendation and privacy protection. There are several directions can be further investigated. First, in this paper, we build our model based on traditional machine learning methods. We would like to study privacy preserving social recommendation with deep learning techniques. Second, in this paper, we only consider static recommender systems. We would like to investigate privacy preserving issue for temporal and dynamic data [17] as well.