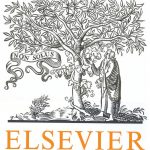
ترجمه مقاله نقش ضروری ارتباطات 6G با چشم انداز صنعت 4.0
- مبلغ: ۸۶,۰۰۰ تومان
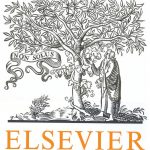
ترجمه مقاله پایداری توسعه شهری، تعدیل ساختار صنعتی و کارایی کاربری زمین
- مبلغ: ۹۱,۰۰۰ تومان
Abstract
Sensitivity analysis establishes priorities for research and allows to identify and rank the most important factors which lead to great improvements in output factors. The aim of this study is to examine sensitivity analysis of inputs in grape production. We are proposing to perform sensitivity analysis using partial rank correlation coefficient (PRCC) which is the most reliable and efficient method, and we apply this for the first time in crop production. This research investigates the use of energy in the vineyard of a semi-arid zone of Iran. Energy use efficiency, energy productivity, specific energy and net energy were calculated. Various artificial neural network (ANN) models were developed to predict grape yield with respect to input energies. ANN models consist of a multilayer perceptron (MLP) with seven neurons in the input layer, one and two hidden layer(s) with different number of neurons, and an output layer with one neuron. Input energies were labor, machinery, chemicals, farmyard manure (FYM), diesel, electricity and water for irrigation. Sensitivity analysis was performed on over 100 samples of parameter space generated by Latin hypercube sampling method, which was then fed to the ANN model to predict the yield for each sample. The PRCC between the predicted yield and each parameter value (input) was used to calculate the sensitivity of the model to each input. Results of sensitivity analysis showed that machinery had the greatest impact on grape yield followed by diesel fuel and labor.
4. Conclusion and direction for future research
Modern crop production requires significant amount of energy. Efficient energy use in agriculture is a necessary step towards decreasing environmental issues and increasing agricultural sustainability. Thus, finding the important factors contributing on crop yield is important. Prediction of crop yield based on energy use is important for farmers, governments, and agribusiness industries. Artificial neural networks a learning machine technique is used to dealing with nonlinear and complex relationships between inputs and output. Therfore, to predict grape yield with respect to input energies, various multi-layer perceptron ANN models were developed with one and two hidden layers. The best ANN model had 7-6-1 topology with high correlation coefficient between predicted values and observed data. Sensitivity analysis of input parameters was determined using partial rank correlation coefficient (PRCC). It showed that machinery had the greatest impact on yield. Therefore, agricultural mechanization is the first priority for increasing grape yield in the studied region. This study can be generalized for semi-arid regions with the same latitude, but the impact of climate change that may affect results, requires further investigation.
In many real applications data reported are not crisp data, hence, future research could focus on including the uncertainty and develop a fuzzy network for the proposed ANN.