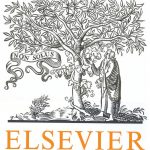
ترجمه مقاله نقش ضروری ارتباطات 6G با چشم انداز صنعت 4.0
- مبلغ: ۸۶,۰۰۰ تومان
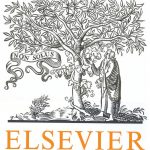
ترجمه مقاله پایداری توسعه شهری، تعدیل ساختار صنعتی و کارایی کاربری زمین
- مبلغ: ۹۱,۰۰۰ تومان
Abstract
Purpose: Data mining is widely considered necessary in many business applications for effective decision making. The importance of business data mining is reflected by the existence of numerous surveys in the literatures focusing on the investigation of related works using data mining techniques for solving specific business problems. However, there has been no recent study answering the following question: What are the widely used data mining techniques in business applications? Design/methodology/approach: The aim of this paper is to examine related surveys in the literature and thus to identify the frequently applied data mining techniques. To ensure the recent relevance and quality of the conclusions, the criterion for selecting related studies are that the works be published in reputed journals within the past 10 years. Findings: There are 33 different data mining techniques employed in eight different application areas. Most of them are supervised learning techniques and the application area where such techniques are most often been is bankruptcy prediction, followed by the areas of customer relationship management, fraud detection, intrusion detection, and recommender systems. Furthermore, the widely used 10 data mining techniques for business applications are the decision tree (including C4.5 and CART), genetic algorithm, k-nearest neighbor, multilayer perceptron neural network, naïve Bayes, and support vector machine as the supervised learning techniques and association rule, expectation maximization, and k-means as the unsupervised learning techniques. Originality/value: The originality of this paper is to survey the recent ten years of related survey and review articles about data mining in business applications in order to identify the most popular techniques.
5. Conclusion
This study investigates the related survey literature in order to identify the frequently used data mining techniques in various business application areas. In total, eight business application areas where data mining techniques are used are surveyed. They are bankruptcy prediction, customer relationship management, fraud detection, intrusion detection, recommender systems, software development effort estimation, stock prediction/investment, and other financial time-series areas.
In addition, it is found that 33 different techniques have been employed in these eight business application areas. Among them, there are 24 and 9 supervised and unsupervised learning techniques, respectively. This demonstrates that most business problems are prediction oriented. Furthermore, bankruptcy prediction is the most widely studied application area where 15 different techniques were used.
For the widely used data mining techniques, 10 different techniques are identified, which include 7 supervised and 3 unsupervised techniques, namely decision trees (including C4.5 and CART), genetic algorithms, k-nearest neighbors, multilayer perceptron neural networks, naïve Bayes, and support vector machines as the supervised learning techniques and association rules, expectation maximization, and k-means as the unsupervised learning techniques.